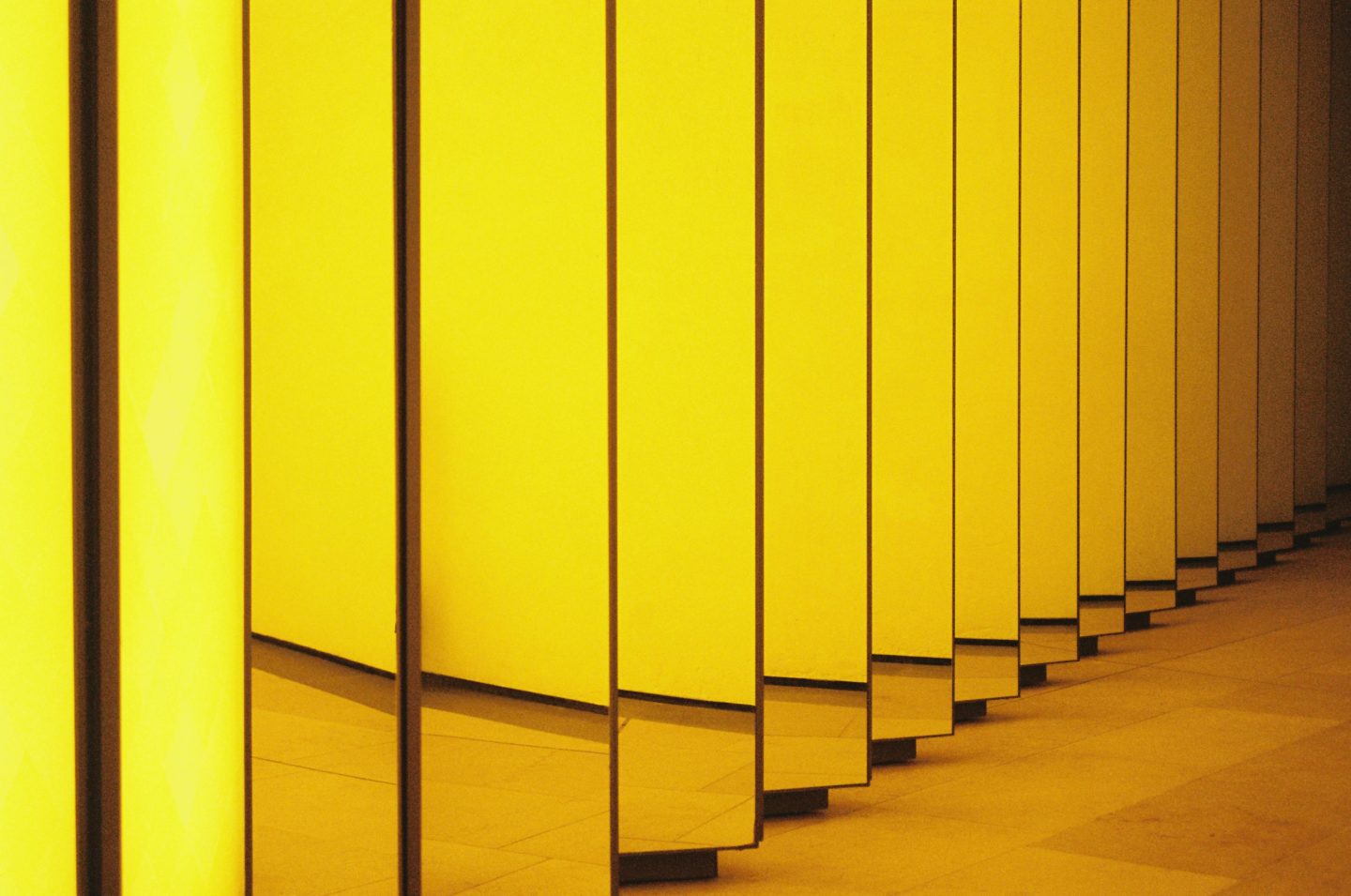
- Artificial Intelligence
- Machine learning
In the survey among U.S. healthcare providers conducted in spring 2021, only 8% of respondents said they were taking system-wide coordinated efforts to implement artificial intelligence (AI) and machine learning (ML).
Should we expect this number to grow in the coming decades? The World Health Organisation says AI and machine learning will enhance health outcomes. Health institutions can apply machine learning in genetic information analysis, natural language processing of medical documents, and medical imaging. Medical image analysis is of particular interest since it can help diagnose cancer, the second deadliest disease in the U.S.
At the moment, histopathologists analyze images of tissues or cells visually. This process is time-consuming and prone to errors. And this is where machine learning can step in and help by automating the process.
Let’s now explore machine learning opportunities in histopathological image analysis.
Today, the healthcare industry generates about 30% of global data. The abundance of data is a source of new opportunities—and challenges, too. To handle the latter, medical organizations and startups can leverage machine learning methods. In particular, ML can lend a hand in disease detection, diagnosis, and prognosis.
Given that 5% of patients in the U.S. are misdiagnosed, and in about 10% of them, misdiagnosis causes death, ML methods can literally save lives by improving accuracy.
The standard clinical practice of assessing a histopathological image (HI) engages trained pathologists. They examine images and carefully assess their colors, shapes, peculiarities, and relationships to make a diagnosis.That is why this task is not error-free and takes up too much time. Machine learning methods can help pathologists automate a large part of their job.
ML detection algorithms can recognize certain histological structures. These are regions of interest that may include lymphocytes, cancer nuclei, and glands. The algorithms go on to identify specific morphological characteristics of the cell. These characteristics help map HI onto the existing disease categories.
The methods of detection and segmentation for histopathological image analysis are also effective for counting objects—usually cells and nuclei. Their number is significant, as its the basis for rating the severity of disease and providing patients with a prognosis.
Machine learning methods can do more than classify images. They also help discover novel objects, rare tumors, and foreign bodies. ML algorithms can find hidden structures—even if there’re no data available about them yet—and, thus, facilitate decision-making.
One-class kernel principal component analysis (KPCA) is one of the widely used algorithms for outlier and novelty detection.
Accurate tissue diagnoses are essential during tumor surgery. Using histopathological analysis at the time of operation is already a challenge, as it has to be done fast. What’s more, the samples used during surgery differ from the ones in non-urgent histopathology. For this reason, there are fewer data to train machine learning algorithms.
One of the research teams has tested a multilayer perceptron (MLP) method for diagnostic prediction during surgery. Regardless of errors, the research shows that MLP can be used for automated intraoperative histopathological image analysis in medical decision-making. There are some other ML methods medical organizations try out in field conditions.
ML methods can be divided into two categories depending on the availability of labeled data. The algorithms that use labeled data are called ‘supervised learning,’ and those using unlabelled data are ‘unsupervised.’
Supervised learning algorithms infer a function that can map new input images onto the appropriate label. These algorithms excel at classification. Given a set of image characteristics, they can identify a cell as cancerous.
The most common supervised algorithms are neural network,support vector machines, random forest, decision tree,k-nearest neighbor, and Naive Bayes.
Unsupervised learning algorithms use unlabeled data and find patterns within a particular dataset. These algorithms are used at clustering data and, thereby, finding hidden structures. One of the most common algorithms is k-means clustering.
Even though there are still roadblocks to the wider adoption of ML in medical image analysis, MedTech companies are actively experimenting with various methods in several medical fields.
Most medical organizations use machine learning methods in computer-assisted diagnosis systems. Here’s a rundown of common use cases of ML methods in pathology.
Computer-Assisted Diagnosis (CAD) uses machine learning methods for histopathological image analysis. CAD helps with histology image analysis for carcinoma detection and grading. In particular, CAD has been used in early breast cancer diagnoses. It provides histopathologists with a reliable second opinion.
CAD helps through all the diagnosis steps. It starts by taking an image with a microscope-fixed digital camera. Next, it helps with preprocessing the image. The system removes extra areas or normalizes color if needed. Then, the system uses supervised machine learning segmentation techniques to analyze a histopathological image and detect regions of interest.
Another step toward the diagnosis is feature extraction. Algorithms identify distinguishing morphological or other features of the tissue. Based on its features, the system identifies the formation as benign or malignant.
Content-Based Image Retrieval (CBIR) is another ML-based system that extracts images similar to a query image. It’s a great fit for histopathology image analysis, where a simple text-based description might be insufficient to describe the image content.
CBIR uses supervised and unsupervised machine learning techniques, like image dimensionality reduction, as well as deep learning techniques. It retrieves the needed images with great accuracy and high speed. Finding a comparable image fast is most helpful in diagnosing rare cases of diseases.
Clinicopathological relationships analysis helps find correlations between the morphological cell features and their clinical behavior, such as histology of stroma in breast cancer. Finding relationships helps grade tumors, plan treatment, and make the prognosis.
Pathologists can choose from a variety of ML methods, but they should be aware of their limitations. Let’s explore major barriers to the wider adoption of ML in medical image analysis.
Getting a histopathological image is complex, as HI is more about textures than objects with well-defined borders. HI has to be interpreted by a pathologist, too. These two limitations are the main source of histopathological image analysis challenges. Other issues include:
Machine learning algorithms need labeled data for their training. There’re plenty of healthcare data overall, but the labeled HI data is still in deficit. It takes time to label data, and only a trained pathologist can do the job. Newly developed computer systems supporting simple image annotation don’t solve the problem, as there are just not enough pathologists. The trouble is real: the number of pathologists in the U.S. dropped by 18% between 2007 and 2017, while their workload spiked by a whopping 42%.
Unstable conditions during image acquisition may result in image variations. Using different staining reagents may impact the color, and some conditions during tissue slicing might cause wrinkles. Sometimes, images get corrupted by dust. This might lead to incorrect interpretations and misleading results.
The task of visualizing an entire tissue image acquired for analysis hits the limits of computing power. If the image is resized, accuracy suffers. Given that, pathologists have to divide the image into smaller patches. However, even if all the patches are classified accurately, the result might have too many false positives.
Machine learning is not used too widely, but successful cases are not that rare. A hospital in Seoul reported that the accuracy of their diagnoses upped from 83% to 87%, thanks to ML. The world’s growing population is expected to boost the demand for these technologies. So it only makes sense for forward-looking organizations and MedTech startups to invest in ML.
Most ML initiatives in healthcare are still in their infancy stages. There are some significant roadblocks to implementing them in histopathology: a lack of labeled data, image variations, and limited computing power, to name just a few.
Despite these barriers, the field is continuing to progress. Machine learning methods of segmentation, detection, and classification are used to diagnose diseases, make prognoses, and plan treatment. The results show that machine learning can improve diagnostic accuracy and efficiency.
Machine learning has exciting prospects in medical images analysis. With the demand for these technologies rising, MedTech companies should seriously consider investing in their research and practice.
If you are looking for ways to leverage machine learning in your solutions, please don’t hesitate to contact us. At Postindustria, we can help you develop your digital health products using the power of ML. Just schedule a session with one of our experts to discuss your product.