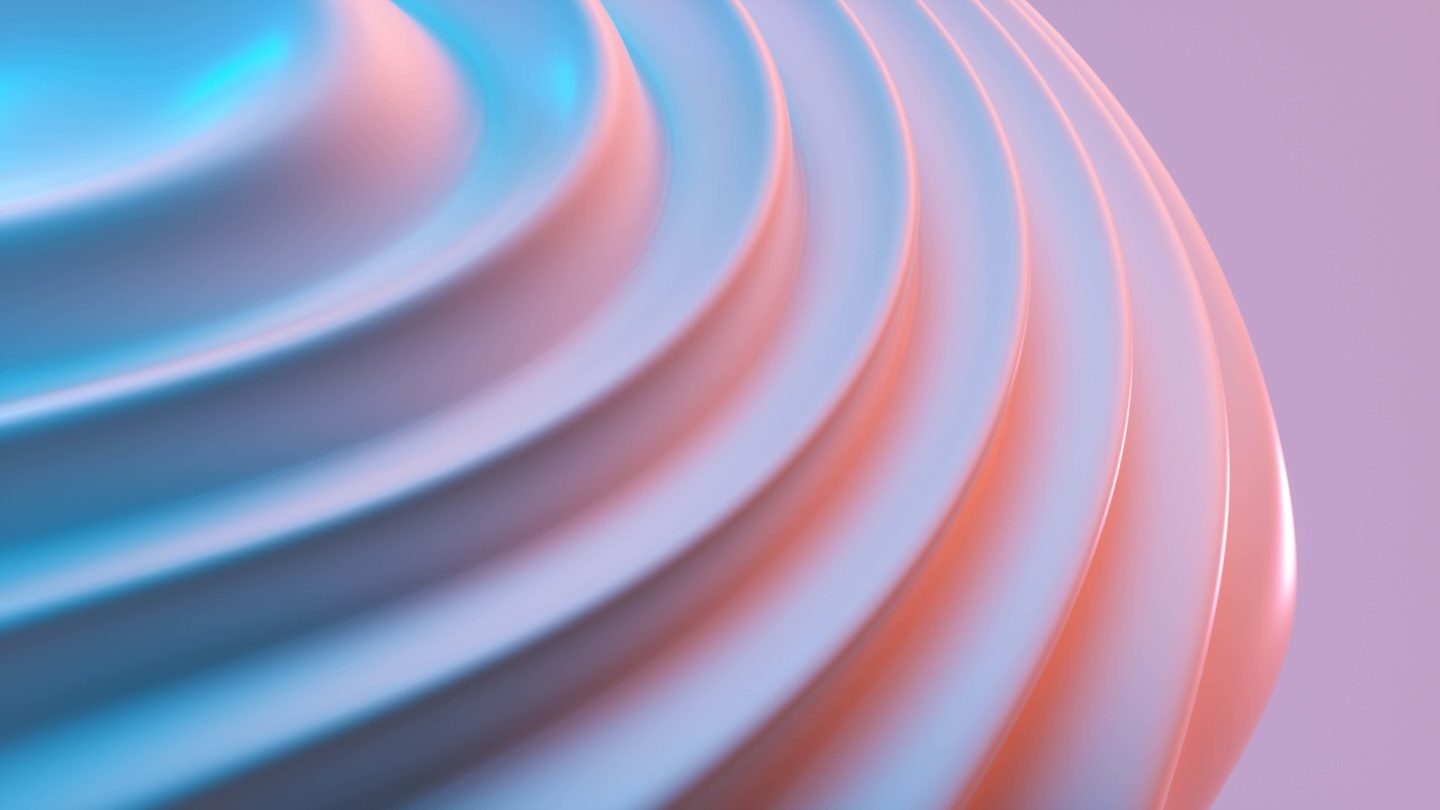
- Artificial Intelligence
Using AI Technology for Fashion Trends Prediction and Demand Forecasting
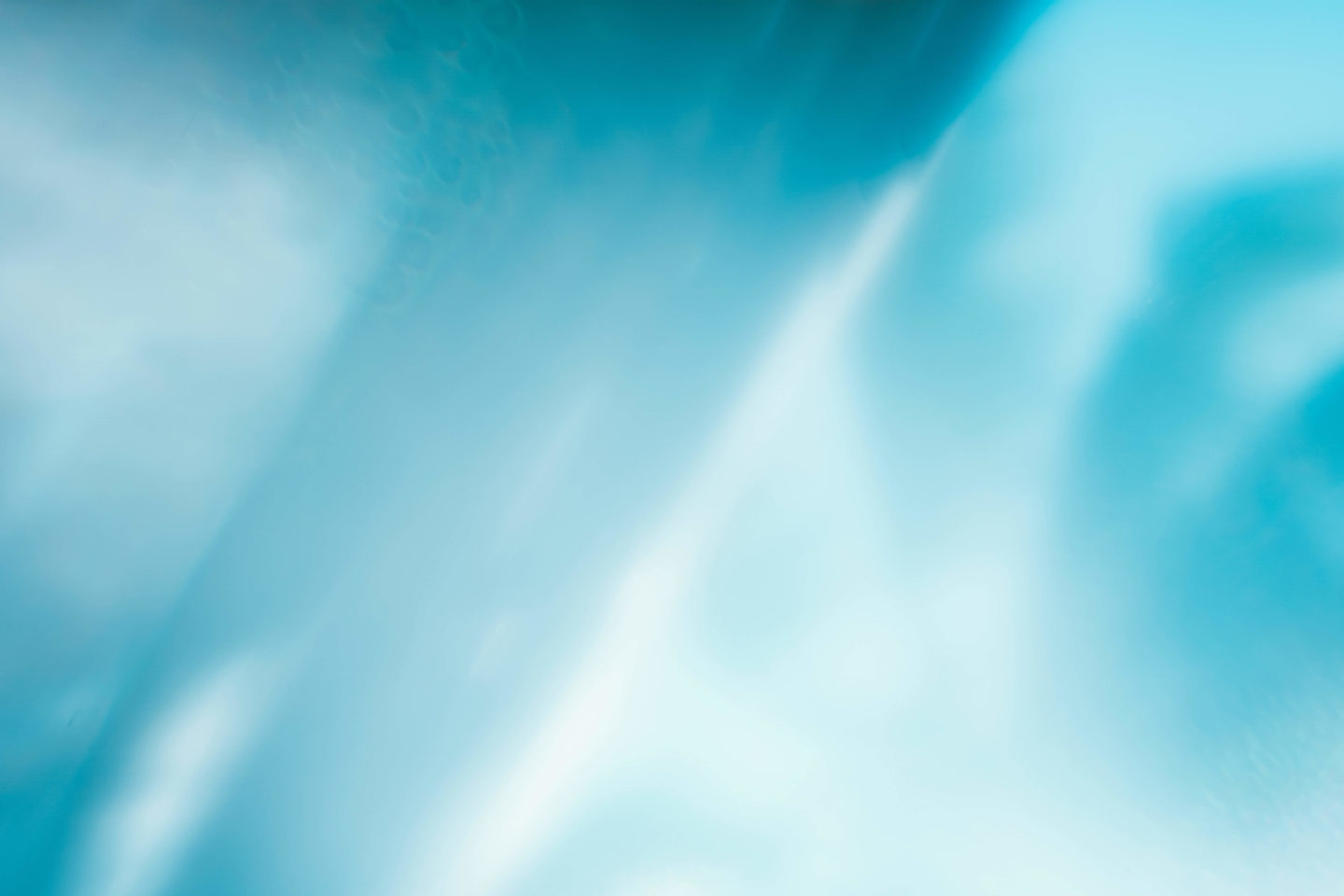
- Artificial Intelligence
AutoML Current Uses and Approaches
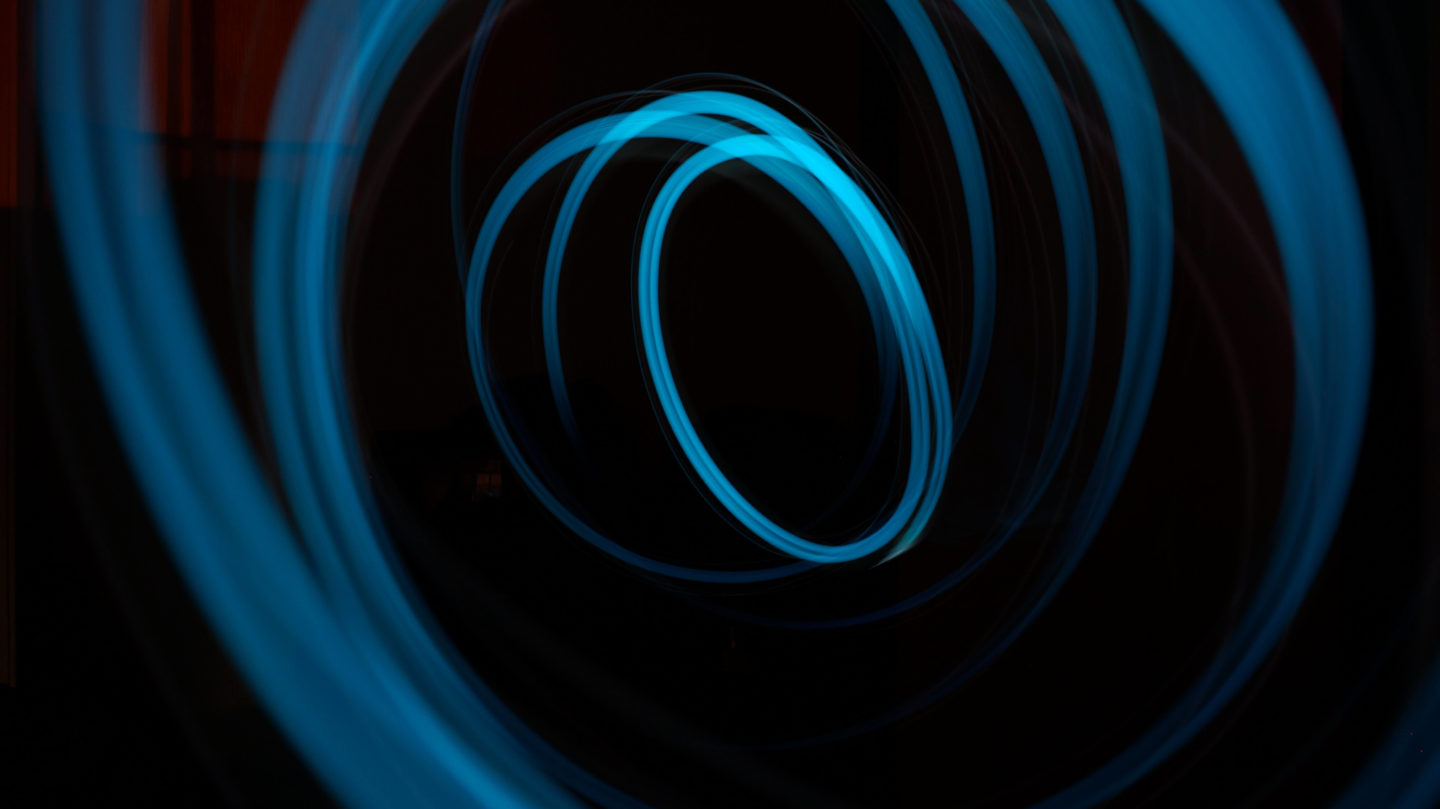
- Artificial Intelligence
Manufacturing production is often prone to disturbances and disruptions. With a system involving so many complex machines and dynamics between them, bottlenecks are inevitable.
Throughput bottlenecks are most common: it’s a term used to describe a clog or challenge in the production process that leads to inefficiency in production. In other words, when a factory produces fewer products than it planned to during a certain period, it deals with throughput bottlenecks.
These bottlenecks appear when there is a breakdown in the production machines or because of human errors or behaviors. Now, it’s not a new issue. Both factory workers and manufacturing management always tried to come up with things to do to remove them quickly or prevent them from occurring.
In this article, we’ll talk about new solutions to remove bottlenecks from factories — in particular, about AI-driven bottleneck analysis in manufacturing and how it helps to detect them early, plan for them, and eliminate them.
Bottlenecks in the output system can result in unscheduled stops, unplanned breaks, or halt the manufacture entirely.
There are short- and long-term bottlenecks. Short-term bottlenecks occur within a production cycle or shift; they hurt the output within said cycle or shift, once. Long-term bottlenecks are recurring, so they impact entire production. If there has been a shutdown and the fabric was left without electricity for half an hour, it’s a short-term bottleneck. If old factory equipment continuously underdelivers — there are blockages, long stop times, etc. — that’s a long-term bottleneck.
Unscheduled stops hurt the profit margin of companies. Downtime costs manufacturers about $50 billion yearly, according to Delloite. This research says that a minute of downtime costs $22k for average manufacturing within the automotive industry. As you imagine, that hurts the bottom line terribly, which is why businesses aim to find out the cause of bottlenecks appearing and remove them.
Bottleneck analysis in lean manufacturing suggests that the most common reasons for them are
Plus, of course, you’re continuing to pay people when machines have stopped — that’s wasted labor. Diagnosing and identifying bottlenecks that bug your factories is the first step to removing them. Now, how can AI help with that?
The deployment of AI in the manufacturing process and supply chain leads to a leaner and smarter manufacturing system. Internet of Things (IoT) system — sensors spread across the plant and tracking everything relevant that’s going on with your machine (relevant in a sense of “may affect the production”) and systems that collect this data — sends data to AI. Let’s talk about how AI is used in manufacturing industry.
At Postindustria, we offer a full range of services to develop AI-enabled solutions that will enhance your business.
Leave us your contact information in the form above, and we will contact you to discuss your project.
Bottleneck identification involves locating the point of constraint in the production. AI can identify recent bottlenecks in the process by looking for patterns in the historical data.
After collecting real-world data, AI experts with domain knowledge in manufacturing choose a bottleneck detection method, pre-process, clean the data, and use a rule-based classification or extraction method to detect anomalies.
The input data might contain durations of blockage and starvations (to calculate the average), active durations (to calculate the average and figure out confidence intervals between them), inter-departure durations, the time it takes for a machine to produce one unit or a series of units, takt time, etc. By analyzing this data, each machine in the factory is classified either as a long-term bottleneck or as a non-bottleneck.
Another example: this case study uses the time series generation method, and then hierarchical clustering to group machines according to their behaviors, e.g. in active durations. Visualization helps experts find machines that act uniquely — in other words, bottlenecks.
Short-term bottlenecks can also be discovered via rule-based classification, but the system of detection, needed, might be more complex. The ML classification for the job would need different input: not event data like active duration, but, for instance, binary states of the machines during these events. Consequently, while engineers can build long-term bottlenecks identification models with data extracted from the machines, models to detect short-term ones would require additional sensor data.
The ability of ML to predict likely bottlenecks makes it indispensable to manufacturers. Now, to find the root cause of the bottlenecks with AI and build appropriate plans to remove them, AI specialists — with assistance from factory employees — build models to classify events in which bottlenecks are detected. This work, for example, uses K-means clustering (an unsupervised ML technique) to visualize clusters of unplanned stops. Then, maintenance practitioners use the insights from this diagnosis to prioritize the most pressing and disruptive bottlenecks.
Apart from making a factory more data-driven and helping employees make sense of the data and utilize it to increase productivity in the short term, AI models also predict bottlenecks in the long term.
AI engineers use various forecasting methods on classified input data from the manufacturer to build predictive models. One group of forecasting methods uses only data extracted from machines and another adds contextual information to the pool. The second type models are more accurate but more time-consuming to build.
The predictive process goes like this.
First, AI detects patterns in historical data, processes these data, and forecasts the predictions using processing results. Statistical tools help capture linear dependencies (when X increases, pressure on the Y increases), while ML tools define non-linear ones.
Then, rule-based classification helps divide machines into bottlenecks and non-bottlenecks (recognizes dynamics in the increase of unplanned stops, for example), and builds forecasts for each machine. An example: this work uses a neural network that processes historical floor data and production plans to detect bottlenecks in real-time and project the captured data in the future.
First and foremost, every complex technology described in the paragraph above aims to solve the downtime issue that cost manufacturers a lot. Insights from AI help to formulate a proactive maintenance schedule and predict the breakdowns, which makes budgeting repairs, logistics activities, etc. easier.
Scheduling and resolving issues might be done manually — by managers and maintenance experts in the factory, — or automatically, again, with the help of prescriptive AI. So, apart from predictive maintenance, AI in manufacturing can help with:
Beyond the above, AI has lots of applications in the production process and can be used to design new products to meet demand.
Sensors are invaluable in AI systems. They monitor pressure, vibration, temperature, sounds, and weight on the floor. Changes in preset conditioning indicate a problem, an anomaly, that’s instantly captured by the algorithms.
Apart from that, sensors can monitor employees’ health and other safety measures like smoke, fire, or a gas leak to ensure a safe working environment.
Sensors are also deployed to monitor the movement of products in the supply chain. They gather data that is useful for future operations and meeting regulatory requirements (e.g. expiration dates, air humidity, etc.).
Let’s summarize how the introduction of AI for manufacturing companies increases efficiency and productivity:
Infusing AI solutions to analyze throughput bottlenecks in manufacturing enables a leaner manufacturing model that is more efficient, cost-effective, and safe. Apart from that, the time employees would otherwise spend on resolving challenges with machines can be used to come up with new approaches to production or new products.
Thank you for reaching out,
User!
Make sure to check for details.