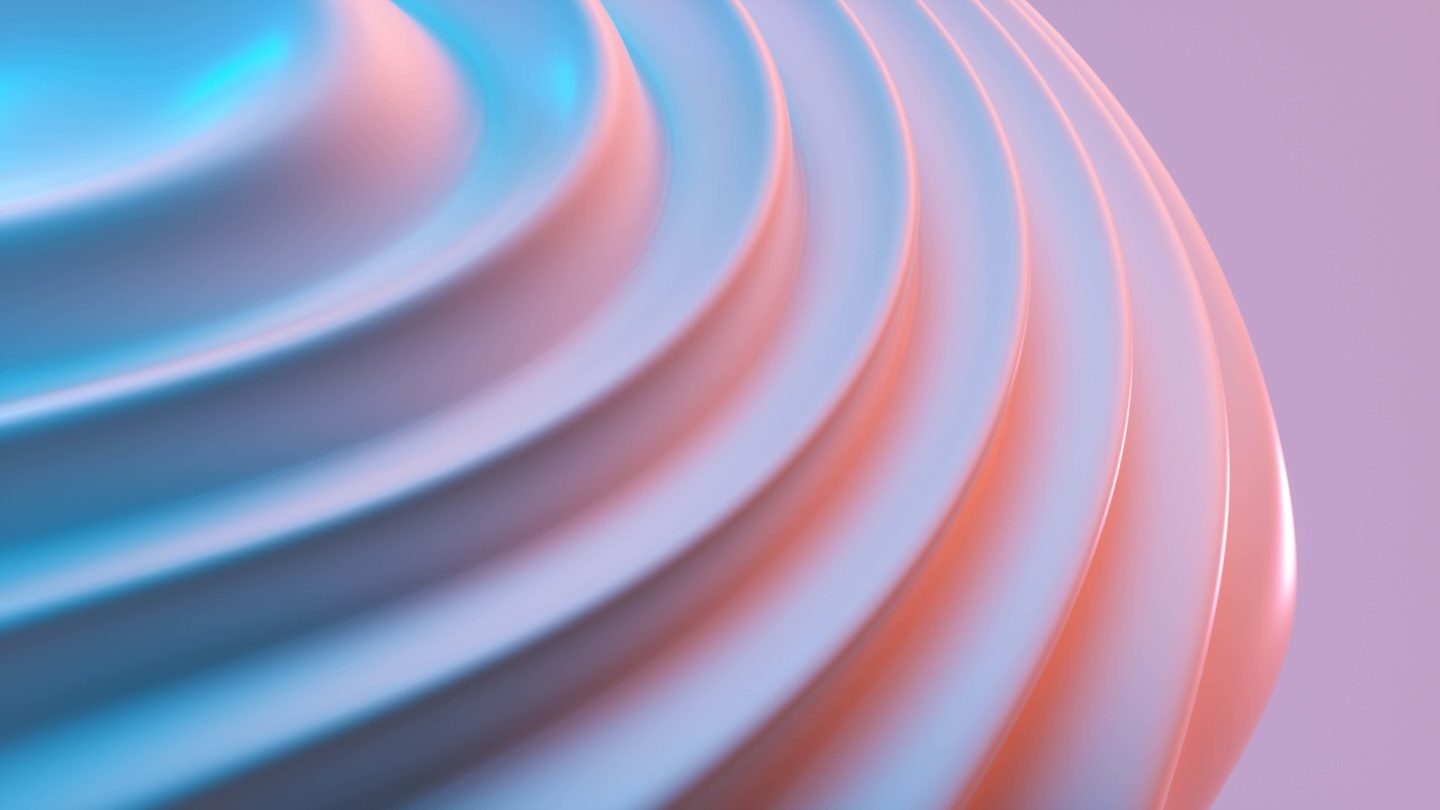
- Artificial Intelligence
Using AI Technology for Fashion Trends Prediction and Demand Forecasting
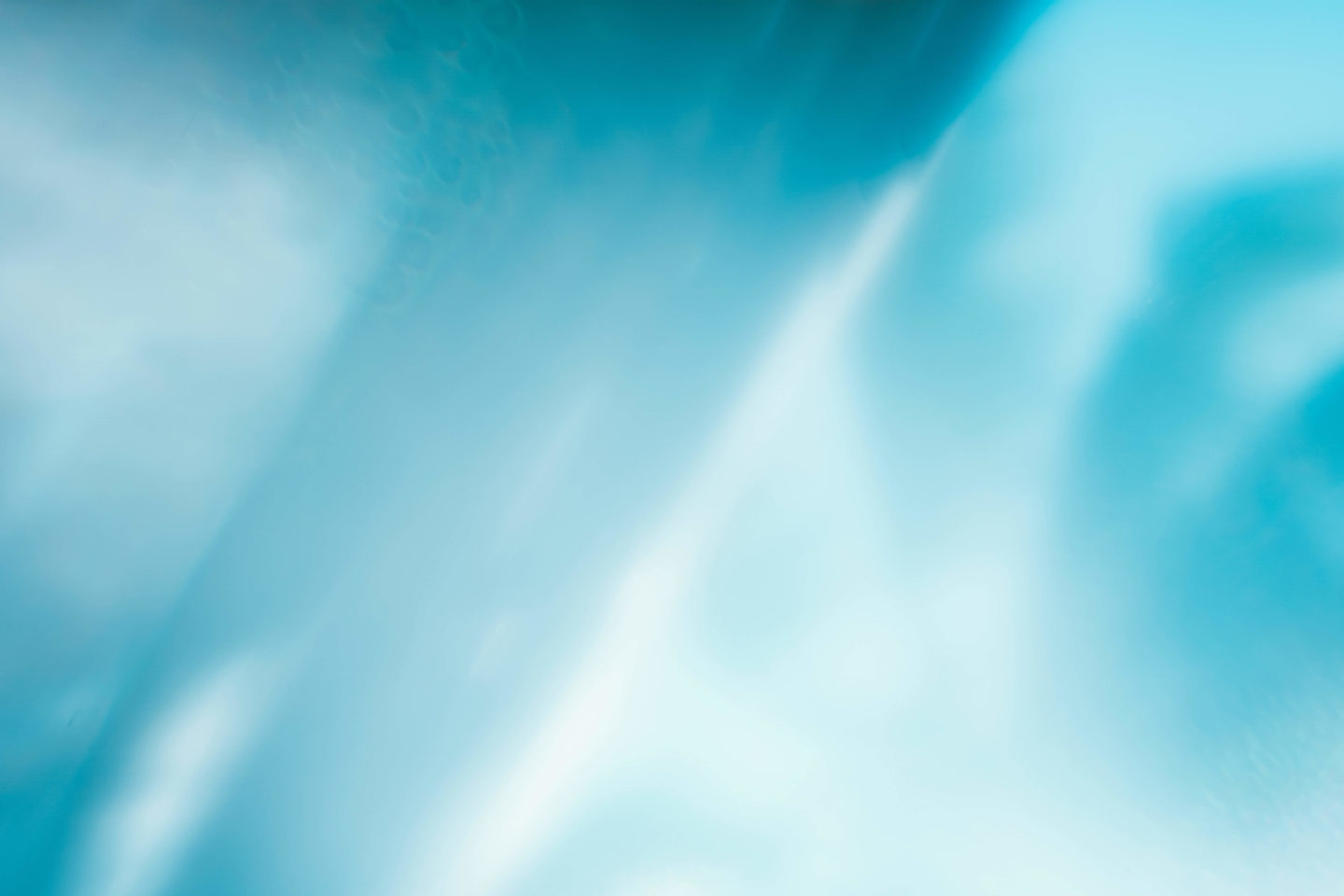
- Artificial Intelligence
AutoML Current Uses and Approaches
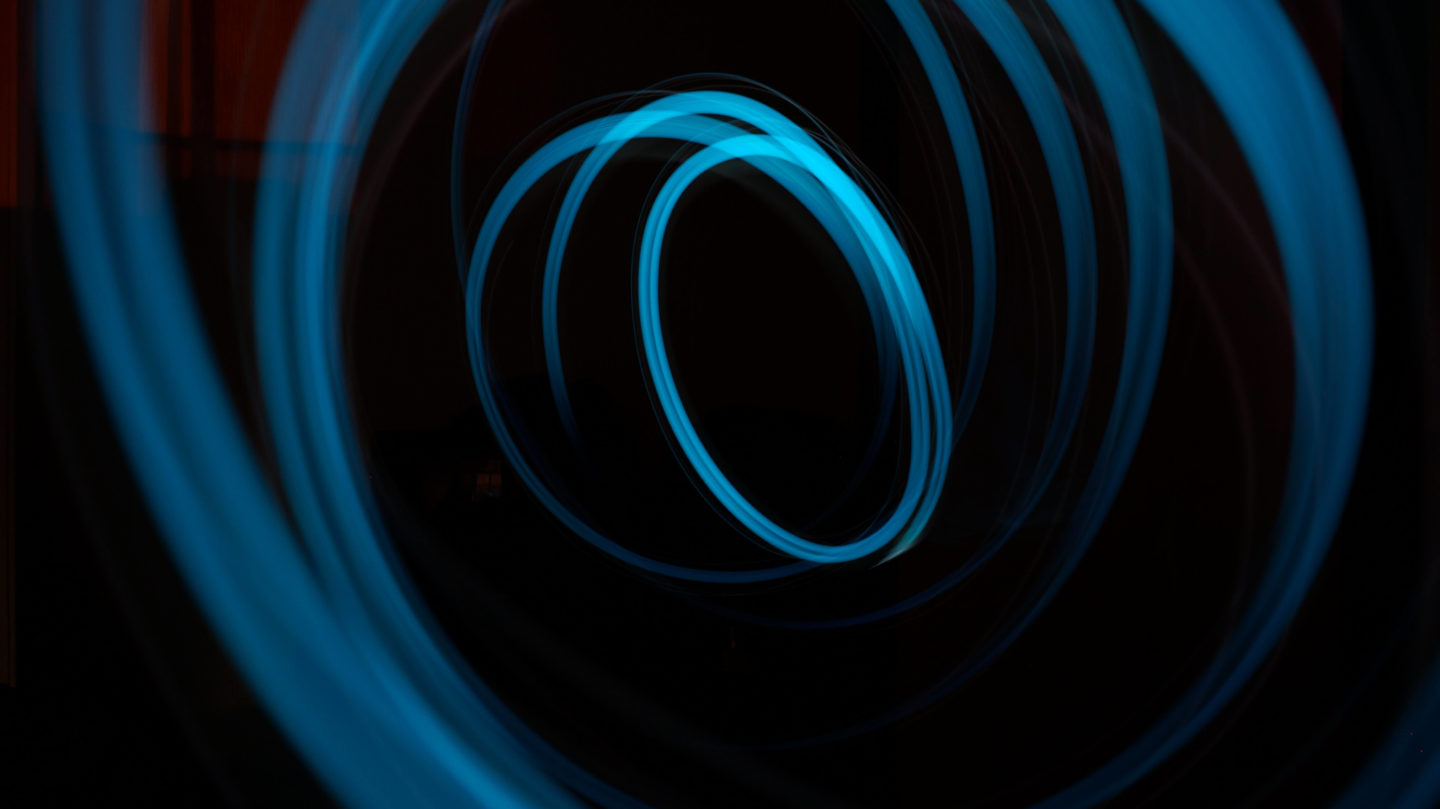
- Artificial Intelligence
The industrial sector is the second largest consumer of energy in the US, with energy consumption accounting for a third of manufacturing’s operational costs. Energy consumption solutions using artificial intelligence (AI) aim to make the manufacturing process more efficient by forecasting energy demand, and in particular, by anticipating machinery usage and predicting malfunction. Energy management software can shift the focus of managers from the daily routine of mundane tasks and monitoring workflow to strategic development.
Energy consumption machine learning (ML) solutions can reduce energy bills by optimizing complex manufacturing processes in any industry. And here’s how.
According to the Energy Information Administration, the industrial sector accounted for almost a quarter of the total energy used in the US in 2020 and 2021. This consumption will only increase. Almost 88% of the energy used in the US comes from nonrenewable sources, and it represents the second largest source of CO2 emissions in the economy, after transportation. Yet up to around half of the energy consumed by the industrial sector is wasted.
There are many reasons for energy waste: breakage, inefficiency, idling machinery drawing electricity, or bulk adjustments in a pharmaceutical plant when the equipment runs without manufacturing. AI can help reduce energy costs, by decreasing waste, optimizing production, and anticipating malfunctions, the last of which helps workers keep up with equipment maintenance before damage occurs. All of this contributes to improving a company’s reputation.
The high price of energy resources and high levels of waste hurt a company’s bottom line. Exorbitant spending on energy and wasted energy shouldn’t be inevitable byproducts of manufacturing. One solution is switching to more renewable energy sources.
Renewable energy has become more affordable recently, making its use more attractive to manufacturers. However, the supply of energy from renewable sources is insufficient to cover industrial demand; therefore, a combination of solutions is needed.
Machine-learning algorithms in manufacturing can create a model of energy demand and usage by learning from historical data and from data collected by sensors and cameras mounted on manufacturing equipment.
After building the model, ML makes predictions, runs hypothetical scenarios, and predicts inefficiencies as they occur so that managers can correct course. AI-driven tools create alerts when predictions differ from a benchmark. This helps minimize or avoid errors in production that can lead to increased energy consumption.
An energy management system must have high-speed smart monitoring and communication to succeed at preventing and resolving errors.
Predictive maintenance aims to improve asset productivity by anticipating machine breakdowns. In an industry that’s highly dependent on machinery, the quicker an operator can repair an issue that triggered an alert, the more they can minimize efficiency losses. Implementing status monitoring can make this happen.
AI-based algorithms can differentiate noise in the data from the important signals to recognize errors and send alerts to maintenance managers, who in turn investigate the issue and make repairs. Thus, predictive maintenance can prevent temporary shutdowns to repair machinery, avoid large operational expenditures, and minimize inefficiencies if, for example, sensors detect an energy leak.
PricewaterhouseCoopers (PWC) reported a case study of how international company 3B-Fiberglass used deep learning at their manufacturing facilities. The two phases of the research consisted of identifying the break and predicting the break in advance. Predicting the break was based on many factors, including temperature, electrical currents, and gas flow. Even though a computer vision algorithm predicted the break without finding the root cause, it helped determine how to minimize breaks, thereby saving costs.
According to a McKinsey Digital report, predictive maintenance can lower inspection costs by up to 25% and maintenance costs by up to 10% by suggesting an asset output schedule for maintenance workers to follow. Predictive maintenance reduces machine downtime, creating more potential savings.
In the manufacturing process, quality testing is another critical stage that impacts energy management. Producing faulty products leads to energy and storage waste. Computer vision algorithms can capture images of products at different stages of manufacturing and compare them to a reference model created by a deep neural network learning algorithm. The deep neural network conducts a visual assessment of good and defective products from different perspectives to create the reference model.
AI can conduct visual quality assessments of a diverse range of products, from machine parts and solar panels to cucumbers in Japan, using an ML-driven framework like TensorFlow. Automating the assessment process allows workers to focus on tackling defects instead of looking for them. McKinsey reports a 50% expected productivity increase, and up to a 90% improvement in defect detection with ML implementation.
Since the Paris Agreement treaty on climate change, there’s been a big move toward a low-carbon economy. The long-term goal is to walk away from fossil fuels. In the short term, this means a continuous transition from nonrenewable sources of energy to renewable ones.
Some energy sources, such as solar and wind, carry the risk of an unstable grid, making manufacturing plants susceptible to blackouts. However, AI can help by predicting capacity levels and creating weather models to ensure an efficient grid. It can also conduct short-term forecasting and control dispatch efficiencies. These models can suggest suitable energy sources to meet energy demands.
In the industrial sector, one of the setbacks for AI implementation is the human factor. Although AI promises a vast number of benefits, a 2018 PwC survey across 26 countries found that out of 1155 manufacturing companies, “only 9% of manufacturing executives have implemented AI”. However, this might indicate a fear of job displacement. AI will not take away work from skilled labor, but rather free them from linear tasks.
“AI is coming for the tasks you hate to do, such as spending lots of time processing data and validating data. The machine can step in and do those mundane tasks, so it augments everyone’s capabilities,” says Phillip Bruner, ENIAN CEO.
Every year AI-driven tools become more user-friendly. Hiring ML/AI teams to enhance local manufacturing expertise with AI software will allow managers to concentrate on repair and strategic questions of optimization, rather than looking for a needle in a haystack.
There are quite a few reports of how combining traditional solutions with AI-driven solutions can achieve greater production efficiency.
An aluminum refinery in Europe collected data from over thirty-two process variables and studied them with the help of statistical techniques and artificial neural networks. They uncovered an overall AI investment payback period of fewer than three years.
The pharmaceutical industry is extremely energy-dependent due to bulk manufacturing, but AI can produce a potential savings of up to 22% in energy bills.
A brewery in Gambia that produces among others, Guinness beer, found an energy-savings potential of 25% with AI-derived low-cost and high-saving solutions for brewing processes and auxiliary systems.
AI solutions can bring a competitive advantage to any manufacturer in any industry because they analyze how to optimize manufacturing processes on their own and combined, thereby minimizing costs while maintaining product quality.
Minimizing breakdowns and prolonging machinery life cycles also decrease costs in the long run, helping businesses to maximize financial returns.
Even though embedding AI into manufacturing is a complex and highly technological process, potential savings are achievable in the short term. Moreover, in the long-term avoiding implementing AI in manufacturing risks falling behind the curve and incurring higher costs than rivals. If you think your business can leverage the power of AI, Postindustria offers a full range of services to deliver solutions based on machine learning. Fill out our contact form and we’ll reach out to discuss your project.
Thank you for reaching out,
User!
Make sure to check for details.