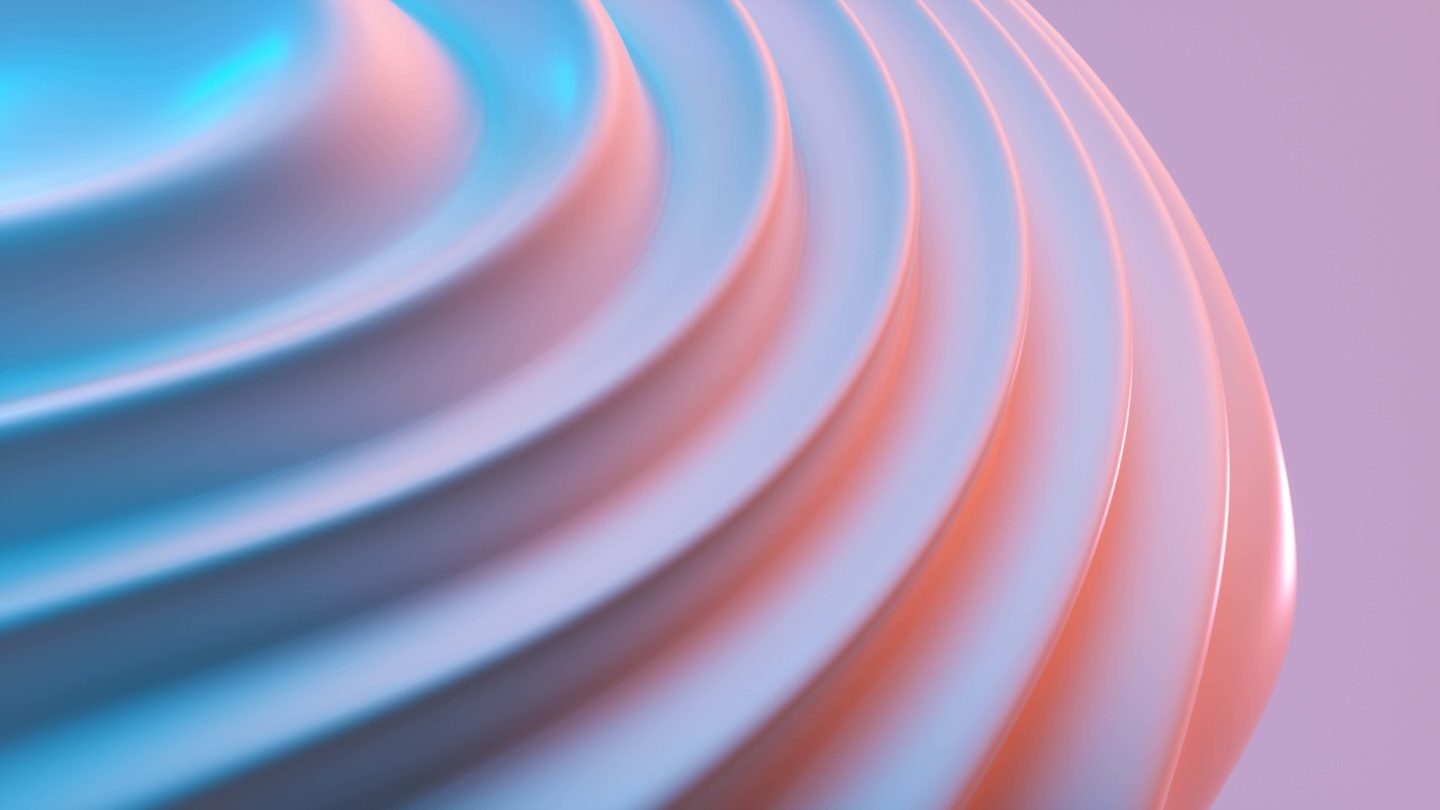
- Artificial Intelligence
Using AI Technology for Fashion Trends Prediction and Demand Forecasting
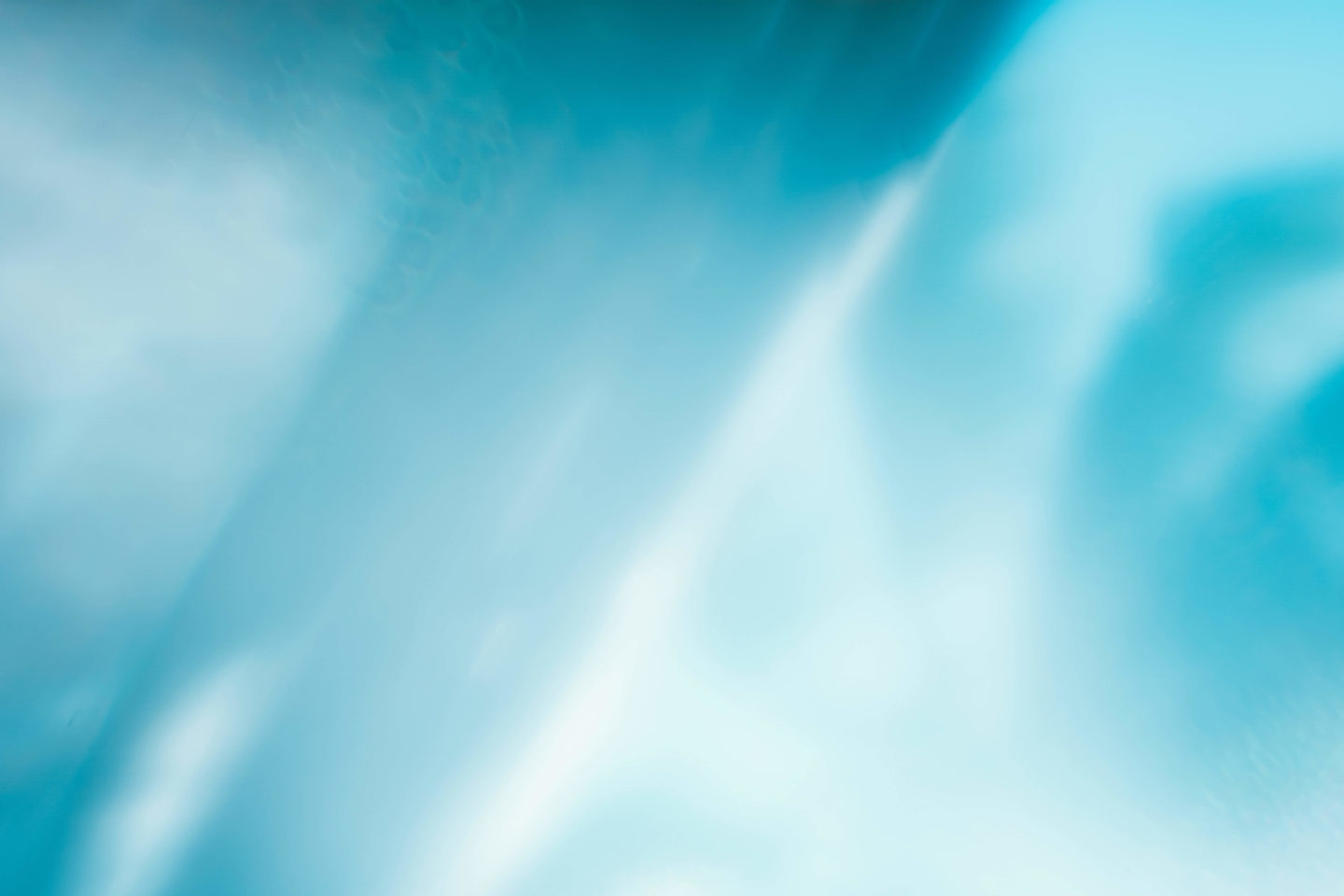
- Artificial Intelligence
AutoML Current Uses and Approaches
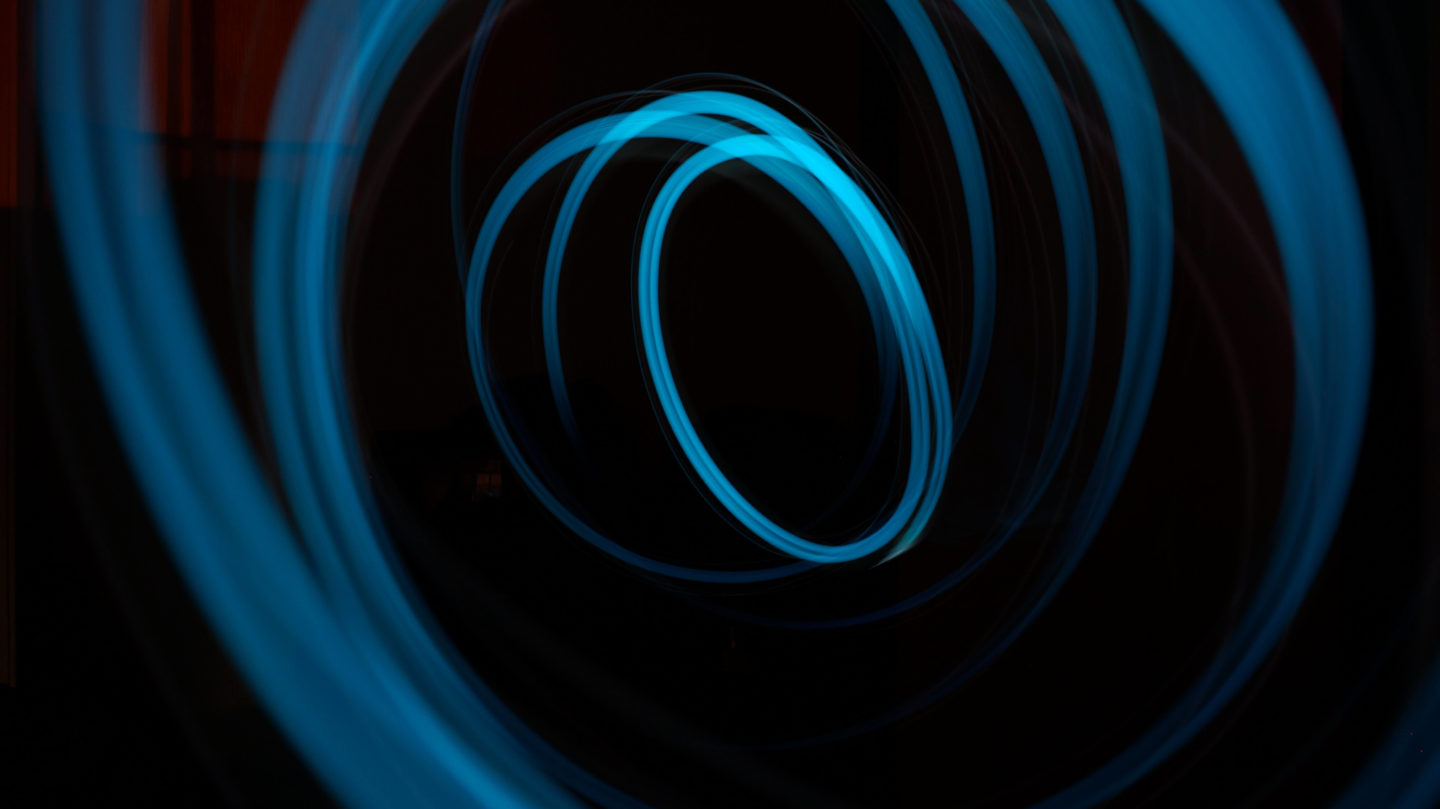
- Artificial Intelligence
According to market research, around a quarter of US adults use smart wearable devices. Driven by consumer demand for monitoring their own health and keeping track of vital signs, the technology’s use has more than tripled in the last four years, Insider Intelligence reports.
Digital medicine with integrated data diagnostics is advancing with wearable trackers that have capabilities far beyond those of the Apple Watch and Google’s Fitbit. Modern wearables can measure blood oxygen saturation levels and monitor glucose in your blood throughout day and night. Some of them, like Lively Wearable for seniors, can be programmed to contact a general practitioner if a patient collapses. Intelligent Asthma Monitoring device forecasts oncoming asthma attacks before the patient even notices any symptoms.
Wearables are also widely used in clinical trials during cancer treatment. These devices are designed for through-the-skin measurement of biomarkers circulating in the blood.
Another field that is heavily reliant on medical data science and big data is electronic health records (EHR) systems. Amassing huge chunks of records about a patient’s health in a digital format, they allow a deeper understanding of disease patterns and improve treatment efficiency.
Despite its potential and adoption advancement, EHR is one of the fields in data-based healthcare that encounter significant roadblocks on their way toward development.
Let’s take a look at some of the biggest challenges clinical data science is facing now.
Privacy concerns
Given the sensitive nature of healthcare records, a data-driven approach to this domain raises well-grounded privacy concerns in the industry and beyond. Leading medical organizations fear that patient data-sharing with apps could facilitate invasions of privacy.
“Patients simply may not realize that their genetic, reproductive health, substance abuse disorder, mental health information can be used in ways that could ultimately limit their access to health insurance, life insurance or even be disclosed to their employers,” Dr.Jesse M. Ehrenfeld, an anesthesiologist who is the chair of the American Medical Association’s board, told The New York Times. “Patient privacy can’t be retrieved once it’s lost,” he added.
The centralization of much of health information also makes the data extremely vulnerable to cyberattacks.
Responsibility issues
When diagnosis is made by a computer program, who takes responsibility for the accuracy of that diagnosis? The difficulty in making this determination is one of the major roadblocks to the advancement of data science in healthcare industry. Although AI has been helping doctors make diagnoses for a while, its accuracy has been far from 100%, and roadblocks data analytics is still needed. However, a recent study by researchers from Babylon Health and University College of London showed that a new algorithm scored higher than 72% of general practitioners in diagnostic accuracy.
This suggests that AI’s accuracy can now exceed that of human doctors. However, this does not solve the problem of liability in the event of misdiagnosis.
Heterogeneity of big data
Regardless of whether you have to deal with clinical data diagnostics or databases in any other domain, you are most likely to encounter information that comes in incompatible formats. This happens because different medical centers use different approaches in the way they measure and record data. Some of these chunks of data can be structured, while others cannot.
According to industry analytics, 80% of data in healthcare is unstructured. That includes images from MRIs, X-Rays, free text, pathology slides, PDF files, and other information from laboratories and monitoring devices. This creates an array of problems with reading information for health experts — from interpreting handwriting to accommodating file sizes.
Another big challenge is that such data is highly error-prone, as some formats turn out to be incompatible with each other.
Interoperability issues
Modern EHR systems lack interoperability, underscoring the need to carry out medical terminology standardization and normalize data to those standards. The thing is that medical record systems were created for individual providers and weren’t meant for exchange. The expansion of the number of record providers and data sources turns databases into collections of disparate low-quality data. This makes additional integration tools necessary.
Algorithmic biases
Another aspect, which needs roadblocks data analytics is algorithmic bias. This is a particular concern for producers of wearable devices since you need to wear sensors continuously for long periods for them to identify vital signs effectively.
Some of the challenges mentioned require government intervention and thoughtful policies that would be able to eliminate privacy concerns. However, policy action should be taken in conjunction with, and not instead of, technical solutions.
Federated machine learning (FML), for instance, might be a solution to the privacy issue. A 2020 peer-reviewed study on FML’s role in facilitating multi-institutional collaborations without sharing patient data showed its superiority over the collaborative learning approach in health data science.
Under this model, all participants benefit from the results without having to request access to other contributing databases. In this case, the so-called black-box nature of the neural network model facilitates security and contributes to privacy as the resulting algorithms will be too abstract to deconstruct.
As for the heterogeneity of big data, despite creating lots of inconveniences for health specialists, it serves as an excellent foundation for training machine learning models that would help generalize medical procedures and treatment protocols across the industry in the future. This means that technology companies can actually leverage this challenge at the current stage.
The same applies to addressing interoperability issues. Machine learning can be effective in sifting through high volumes of low-quality data.
Speaking of algorithmic biases, in wearables, they can be eliminated with the development of lightweight sensors with improved battery life that will allow users to carry them for longer periods with more comfort.
The advancement of big data has elevated the healthcare industry to a new level. Although it is still up against serious challenges, more expert research and investment in new data-driven solutions will help overcome the obstacles that data science in healthcare industry is facing.
Thank you for reaching out,
User!
Make sure to check for details.