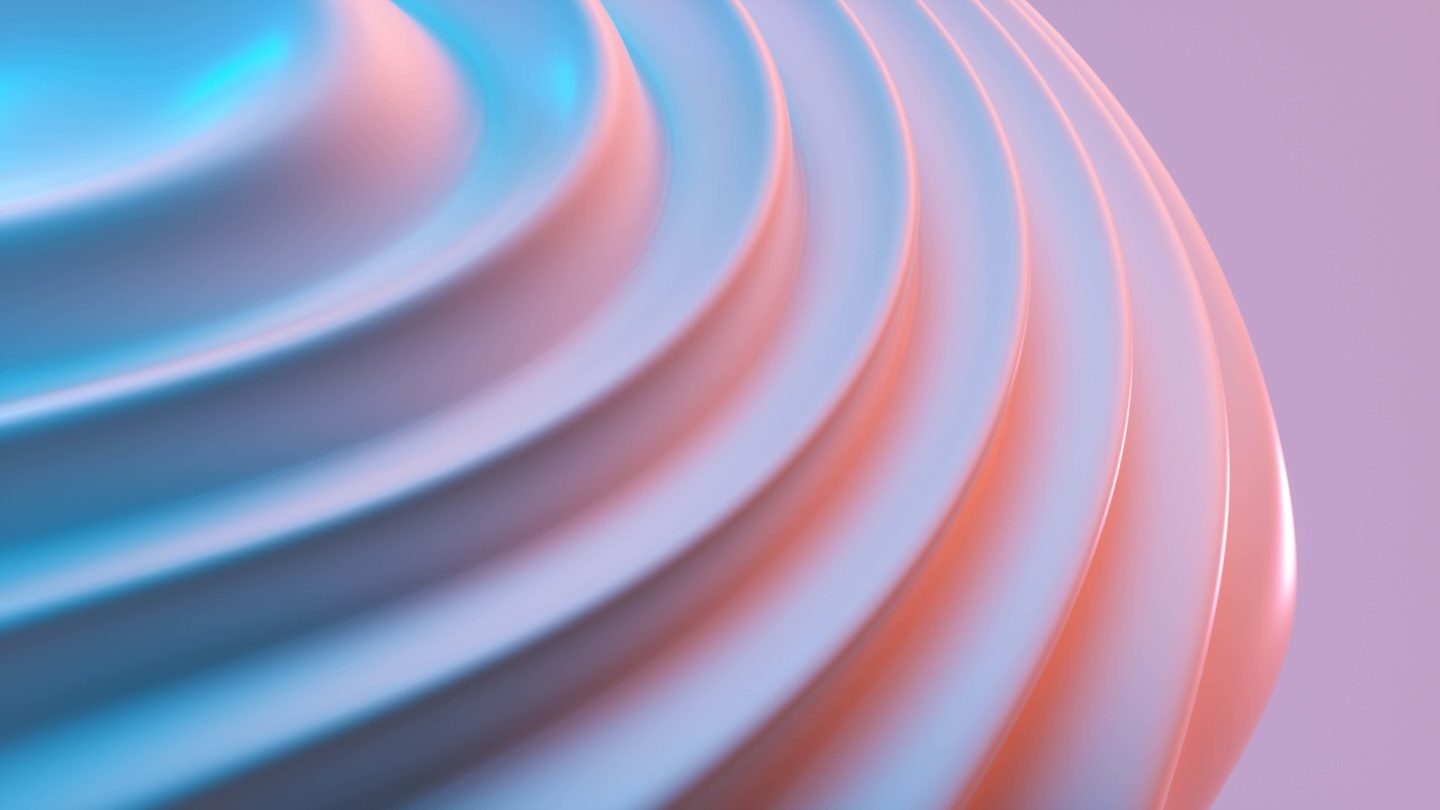
- Artificial Intelligence
Using AI Technology for Fashion Trends Prediction and Demand Forecasting
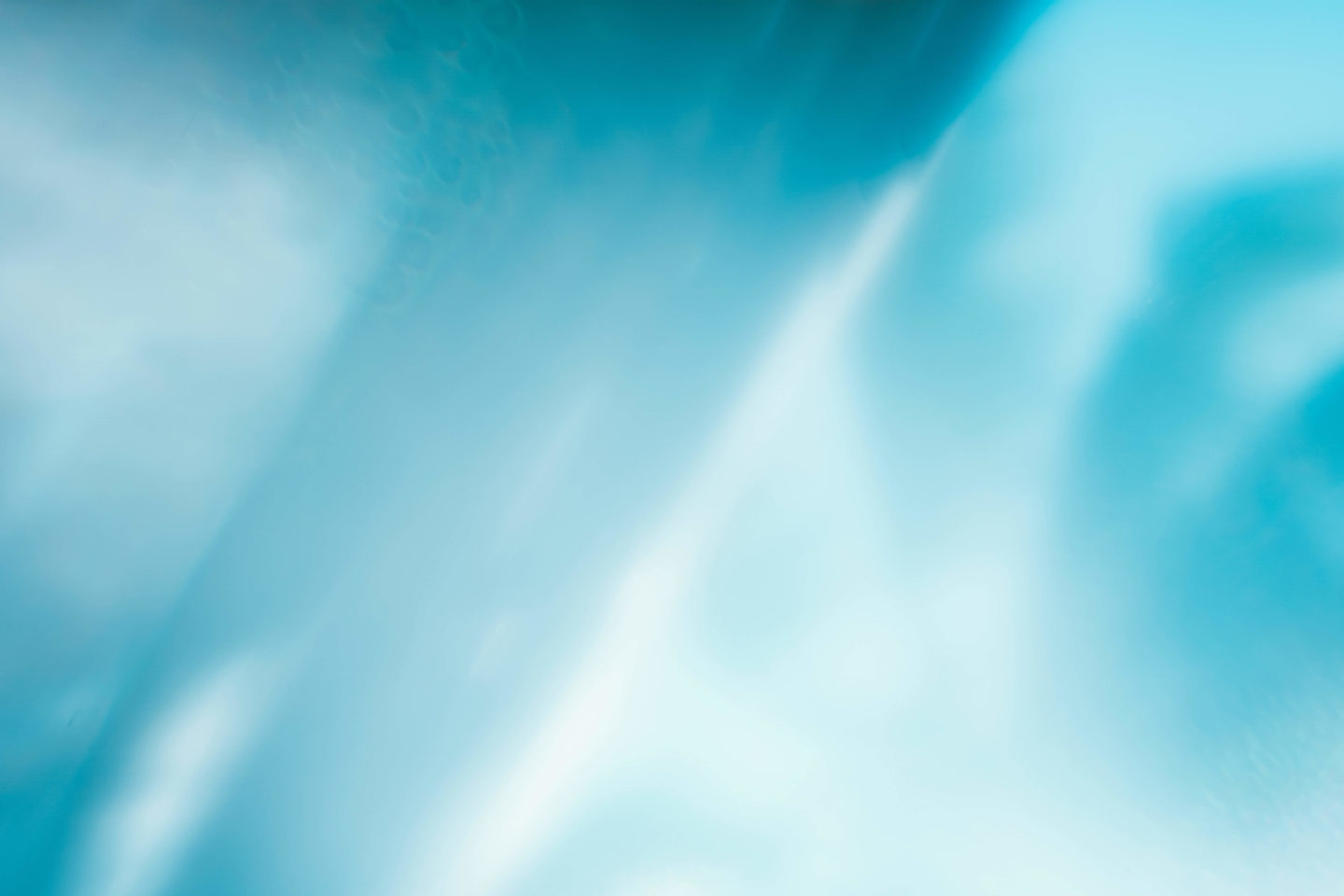
- Artificial Intelligence
AutoML Current Uses and Approaches
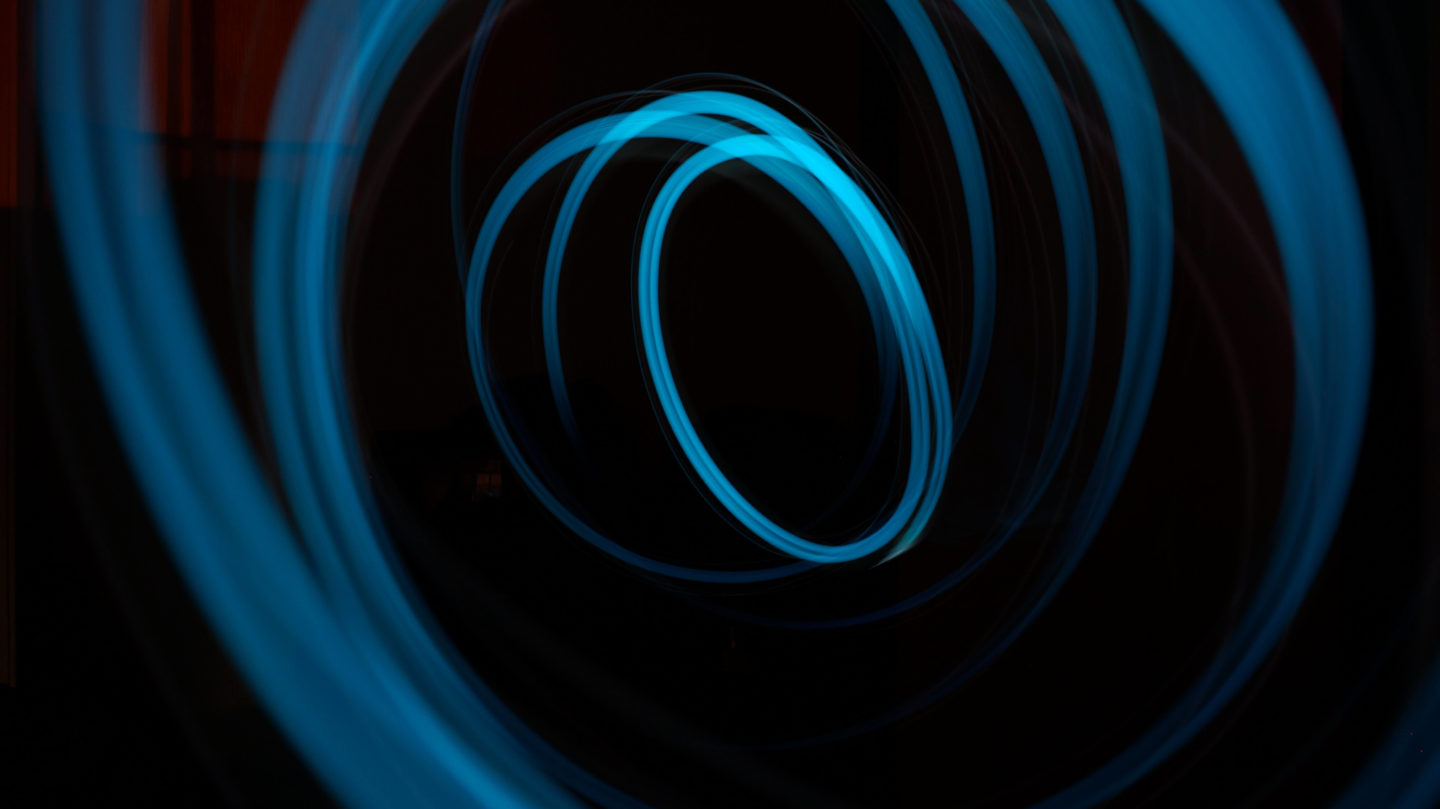
- Artificial Intelligence
Predictive maintenance (PdM) is a life-saver for many manufacturers as it can prevent equipment breakdowns with well-timed repairs and material renewals. Similar to healthcare, prevention in manufacturing is better than cure. But, to maximize the efficiency of PdM, you need to properly implement artificial intelligence and the internet of things into the process. Read along to learn about the benefits of predictive maintenance using AI and how exactly it can help your manufacturing business.
Before we dive into the inner workings of PdM using AI, let’s look at relevant statistics that prove its value.
According to Deloitte, predictive maintenance leads to:
Such impressive stats aren’t surprising as PdM addresses equipment failures before they occur to minimize downtime and breakdowns. But, the proper maintenance is a fine-tuned system that implements the power of both AI and IoT.
Predictive maintenance is a practice for determining the condition of in-service equipment to estimate when maintenance should be carried out. It relies on the data collected by the equipment sensors and its further analysis. So, maintenance is a complex process that poses a few challenges engineers need to overcome to reach its full potential.
Predictive maintenance challenges include:
PdM needs up-to-date and accurate data to function correctly. Even tiny deviations in data’s timeliness and accuracy can result in catastrophic equipment failures due to late maintenance.
The collected data should be transformed into weighty information. It means combining massive amounts of data to find, categorize, and implement its useful parts.
The predictive maintenance framework should be adjusted according to the varying production load, changes in the environment, and new machinery in the production line. So, constant monitoring and information analysis are required.
Fortunately, AI and IoT help overcome the challenges and maximize the benefits of the practice. Here’s how AI-enhanced predictive maintenance works.
With IoT, you can combine various sensors into a unified system that constantly monitors equipment and gathers required data. For example, AI-enhanced PdM can closely monitor
For example, predictive maintenance can use vibration sensors to determine unusual spikes in the vibration frequency and alert personnel that the repair is due.
You can add any other relevant sensor to the IoT system as long as the sensor can interface with the equipment.
Next, the system has to make sense of all the data for predictive maintenance. Here, machine learning algorithms come into play. Learning on massive amounts of training data results in a mathematical model that can sort through the noise and analyze the equipment’s state. As a result, specialized algorithms can react to the unusual changes of crucial measurements and fluctuations to determine deviant behavior and provide a system status or a prediction.
For instance, let’s assume we have a manufacturer that uses big ovens in the production process. We can train an algorithm to predict the oven’s temperature at a given time to avoid overheating and getting out of order. This can be achieved with temperature-tracking sensors and training a predictive maintenance algorithm using regression analysis — estimating the relationship between a dependent variable and independent variables.
With the ML’s output, engineers can react to any otherwise unpredictable changes and adapt maintenance practices on the fly, as well as start repairs before the production line grinds to a halt and a catastrophic breakdown occurs. As far as sensors and ML algorithms work 24/7, the engineers are always up to date on the supply chain status.
As you see, only proper implementation of IoT and ML can help you maximize opportunities. It’s a costly process, but predictive maintenance with artificial intelligence offers plenty of business benefits.
ML in the production line maintenance can benefit business and production processes by providing you with:
Well-trained algorithms for predictive maintenance can foresee future breakdowns with pinpoint accuracy, which saves a lot of time for your engineers and analysts. So, with machine learning, your engineers can switch to creative work and look for new ways to improve the manufacturing processes. Meanwhile, the computers will be busy doing mind-numbing calculations and data analysis.
In conjunction with IoT, ML can keep you updated on the status of the equipment in real-time without exhaustive audits and checks that can take dozens of work hours to complete.
The algorithms don’t suggest changing the oil without the need or buying new parts when the old ones are still in good shape. Also, predictive maintenance with AI shows you how many new parts you need and when you need them. As a result, warehouse management becomes more accessible.
ML algorithms for predictive maintenance are dispassionate. While an independent auditor can tweak the examination results to sell you something, computers will report on the state of your machinery as it is, which can save you thousands of dollars.
With ML, it’s easier to scale your manufacturing capacity by adding new equipment to the production line or new production lines. You just need to apply the same sensor system and algorithms to a new line or add sensors to new equipment and retrain your predictive maintenance machine learning on a new dataset.
All in all, the implementation of ML and IoT in your supply chain is about staying competitive in the modern innovation-driven industry. With proper digitization in place, you can keep your prices down while maintaining the quality of the products at a high level.
Here are a few cases of businesses leveraging AI-enhanced predictive maintenance to boost their productivity.
ZF Friedrichshafen — an automotive industry supplier with 150,000 employees — partnered with Microsoft to implement AI into its production line. In particular, the company focused on predictive maintenance for manufacturing of gears honing process. The challenge was to replace the part of a honing machine — a honing ring — just before the end of its life to prevent it from fracturing during operations and maximize cost-effectiveness.
As a result, the partners developed an AI algorithm that can analyze a wide range of frequencies obtained from honing machines to predict the probability of the honing ring failing. The final solution detected 99% of honing ring fractures before they had occurred.
A chemical facility in the Netherlands, Chemelot, installed sensors on one of its critical factory filters and implemented an algorithm that can predict filter failure and notify of the need to replace it. Overall, the company contributed 60 million euros of annual turnover to its maintenance practices.
Belgian state-owned rail infrastructure company experienced growing pressure of maintaining its 3,600 km of railways in top-notch shape. To address the issue, Infrabel implemented “innovative condition monitoring tools such as sophisticated measurement trains for inspecting tracks, railway ties, and overhead lines; cameras mounted on overpasses to monitor the pantographs of passing trains; sensors for detecting overheating in shaft sleeves on passing trains,” and others.
Using the data, Infrabel keeps its tracks in good shape and quickly replaces any weak parts. The next step for the company is to implement a network-wide AI that will analyze the data and provide real-time insights into the railway conditions.
An international delivery service founded in Germany, DHL Express developed a system that predicts air freight delays and speedups up to a week in advance. It’s not exactly predictive maintenance in general understanding, but the idea is the same: the system analyses data to predict unusual downtimes.
Overall, the bigger the manufacturer, the more benefits they will get from PdM due to the production line complexity.
AI-enhanced PdM can be a game-changer for a big business, boosting output, slashing downtime, and ensuring timely repairs that prolong equipment life. Though the launch of AI-based maintenance requires sizable investments, its long-term benefits compensate initial costs and boost the company’s sustainability. Investing in the predictive maintenance framework will save your engineers a lot of time, avoid unnecessary costs, improve warehouse management, increase your competitive strengths, and get a flexible and scalable production line.
If you think your business can use the power of AI, Postindustria offers a full range of services for the delivery of solutions based on machine learning. Leave us your contact information in the form, and we’ll contact you to discuss your project.
Thank you for reaching out,
User!
Make sure to check for details.