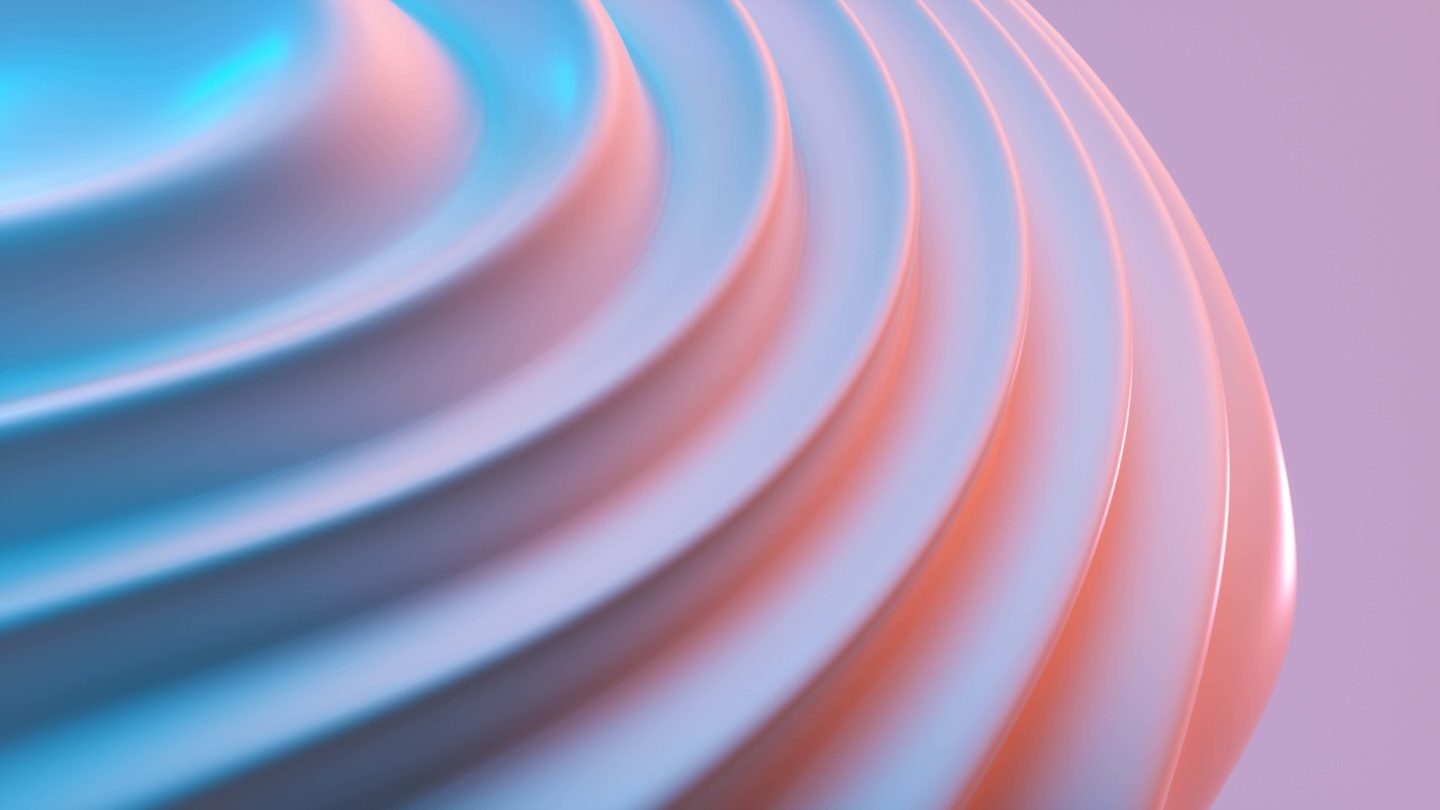
- Artificial Intelligence
Using AI Technology for Fashion Trends Prediction and Demand Forecasting
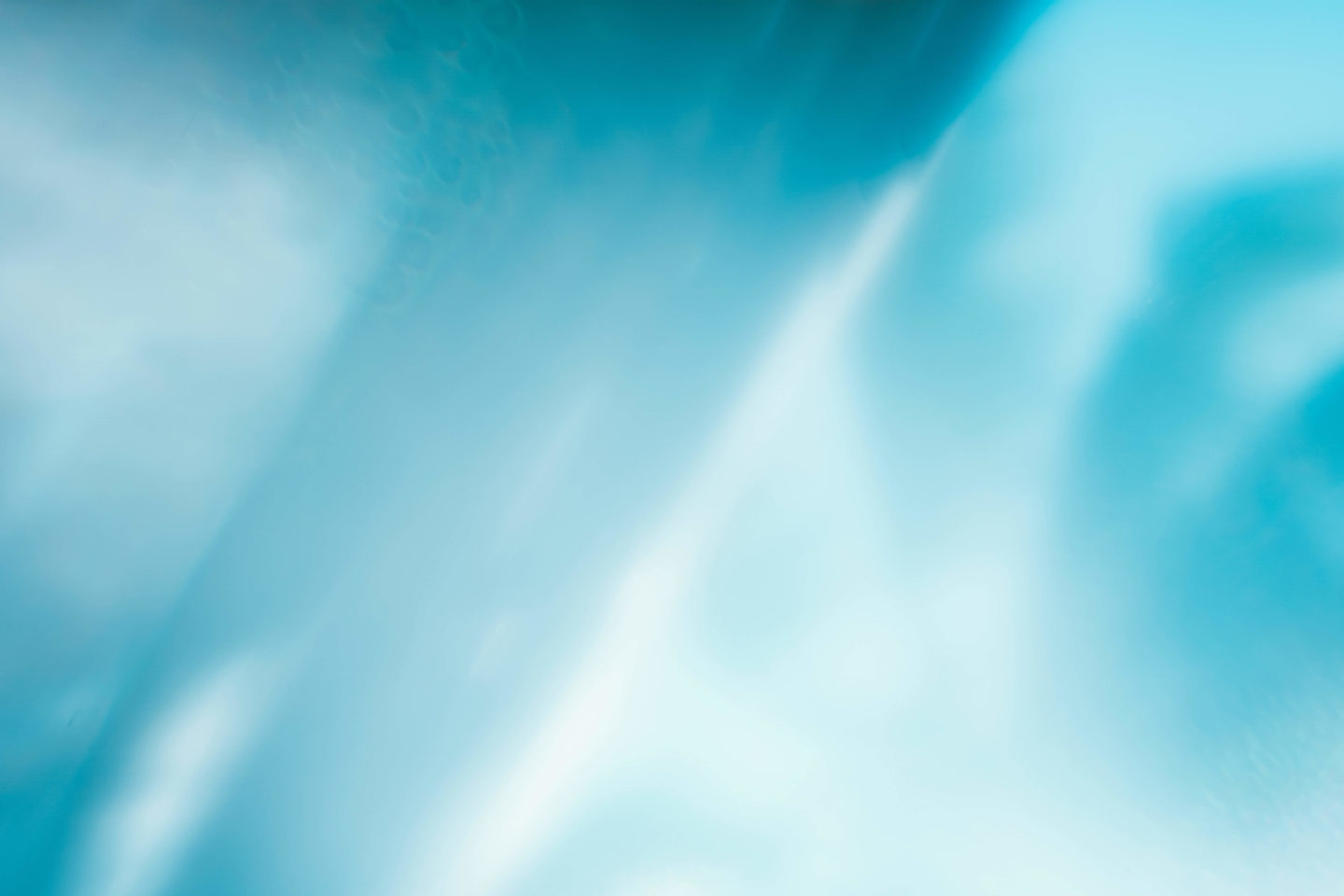
- Artificial Intelligence
AutoML Current Uses and Approaches
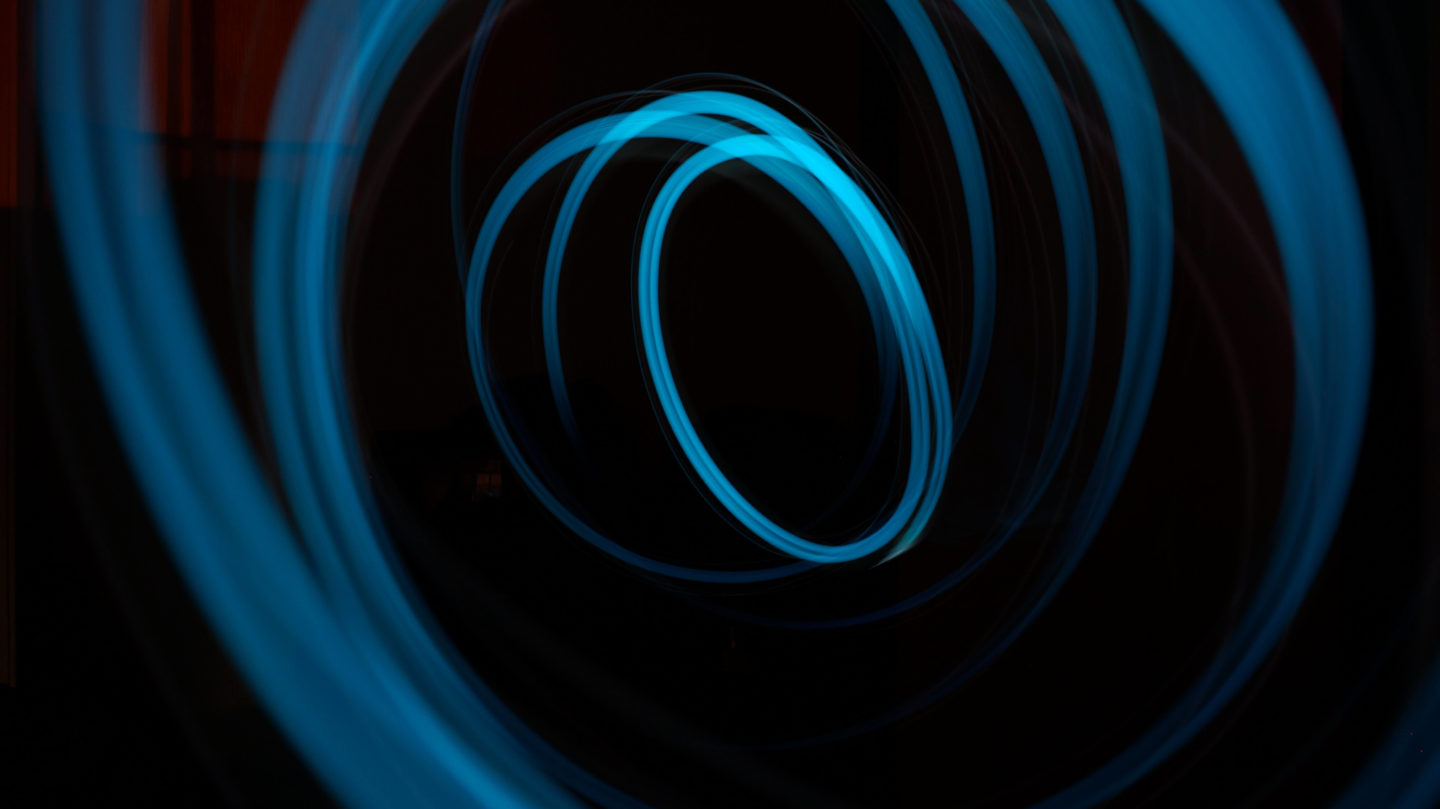
- Artificial Intelligence
Artificial intelligence (AI) can improve the efficiency and effectiveness of treatments in clinical healthcare settings. However, it’s important to remember that algorithms are trained on insufficiently diverse data, which can lead to data bias in AI. With medical centers incorporating more and more technical innovation that incorporates AI, this bias can inadvertently contribute to increasing healthcare disparities.
In healthcare, data bias poses serious risks for patients. For this reason, AI algorithms can either deliver on the promise of democratizing healthcare or exacerbate inequalities. And both are happening today. However, the good news is that the application of AI in healthcare is entirely within our control.
The application of AI to medicine, such as medical imaging, diagnostics, and surgery, will change the relationship between patients and doctors and is set to improve patient outcomes. Algorithms are already doing the most superficial work for doctors, giving them more time to draw up an individual treatment plan for each patient. But AI can be biased.
What is bias in artificial intelligence? People tend to believe in decisions made by computers. People assume that whatever outcome an AI algorithm produces is objective and impartial. However, the output of any AI algorithm is shaped by its input data. When people select the input data for an algorithm, human biases can surface unintentionally.
Today’s world is battling systematic bias in mainstream social institutions, and healthcare centers need technologies that will reduce health inequalities rather than exacerbate them.
Biases can arise at any stage in the development and deployment of AI. For example, the datasets selected to train an algorithm can introduce bias, as can applying an algorithm in contexts other than those for which it was originally trained. We’ll explore these concepts more in the next section.
The most common source of data bias in AI is input information that doesn’t sufficiently represent the target population. This can have adverse effects on the target population. In practice, evidence suggests there is a great deal of bias in technology and AI. Let’s look at four major examples of data bias.
One example of racial inequality in the healthcare industry is a study published in The New England Journal of Medicine in 2020. It caused a stir in the medical community by exposing racial bias in pulse oximetry sensors. The authors found that Black patients were significantly more susceptible to hypoxemia than their white counterparts, despite having comparable pulse oximeter readings. The oximeters did not accurately detect low blood oxygenation in Black patients, which could result in reduced oxygen therapy, thus, increased risk for hypoxemia. Given the prevalence of hypoxemia in COVID-19 patients, this research represents a particularly relevant example of data bias.
As another example, many skin image analysis algorithms have been trained on images of white patients. Since historically, less money has been spent on Black patients with the same level of needs as their white counterparts, the algorithm erroneously assigned Black patients to the same level of risk as healthier white patients. Although now the algorithms are used much more widely for diagnosis in non-white populations, they can potentially overlook malignant melanomas in people with any other skin color.
In the future, AI algorithms that analyze radiological images faster and with more accuracy than humans are expected to increase radiologists’ efficiency and take over some of their responsibilities. But the AI can provide inaccurate analyses due to biased input data.
Some diseases manifest differently in women and men, be it cardiovascular disease, diabetes, or mental disorders, such as depression and autism. If algorithms fail to account for sex differences, care inequalities between sexes can be exacerbated. Therefore, AI algorithms need to be trained using datasets drawn from different populations. However, this is not yet happening.
Socioeconomic status (SES) affects people’s health and the care they receive. For example, people with lower SES are more likely to have poorer health, lower life expectancy, and a higher incidence of chronic disease. Moreover, fewer diagnostic tests and fewer drugs are available to lower SES populations with chronic disease. This population also has limited access to health care due to the cost of insurance coverage and its lack.
Medical practitioners’ implicit bias related to SES leads to inequalities in health care. Data are collected from private clinics, where there are almost no patients with low SES. Thus, cases and possible unique symptoms for patients with lower SES are lost.
A team at the University of Toronto used an artificial intelligence algorithm to identify language disorders that may be an early sign of Alzheimer’s disease. This technology should have made diagnosis easier. However, this algorithm was trained with speech samples from Canadian English speakers, and in practice, it turned out to be useful for identifying language disorders only in speakers of Canadian English. This put Canadian French speakers and those using other English dialects at a disadvantage when it came to diagnosing Alzheimer’s disease.
AI is capable of understanding human language — but which language? The simple answer is the language or dialect it was taught. Unfortunately, this creates a bias that patients and healthcare providers must guard against.
All four examples above show how bias can become ingrained in an algorithm, either due to bias in the selection of research subjects from whom data is collected for training datasets, or due to inappropriate selection of features for the algorithm to be trained on. However, there are also many situations where clinicians themselves introduce bias in algorithms, such as the example of SES above, or by the interaction of clinicians with algorithms.
Although it’s clear that AI bias in healthcare is a problem, this problem is difficult to overcome. It is not enough to simply have a dataset that represents the patient population that you plan to analyze with an algorithm.
We need to understand that in designing an algorithm, we naturally insert our own way of thinking. If we can select data and train algorithms in a way that actually erases the biases that human thinking can introduce, it is possible to gain greater objectivity through AI.
Are there any proven ways to tackle data bias in artificial intelligence? First, we must remember that bias can appear at any stage in the algorithm creation process, from research design, data collection, algorithm design, model selection, and implementation, to the dissemination of results.
Thus, combating bias requires that teams working on a given algorithm include professionals with different backgrounds and perspectives, including doctors, and not just data scientists with a technical understanding of AI.
The sharing of medical data should become more commonplace. But the sanctity of medical data and the strength of privacy laws create solid incentives for data protection and severe consequences for privacy breaches.
There will always be a certain degree of bias because injustice in society affects who can create algorithms and how they are used. Therefore, it will take time to establish normative action and collaboration between government, academia, and civil society. At the same time, we must think about vulnerable groups of people and work to protect them.
Today, more healthcare professionals are at least aware of dataset-related bias in AI. Many companies are taking active steps to promote diversity, fairness, and inclusion in their teams.
Sometimes even when institutions want to share data, a lack of interoperability between medical record systems remains a significant technical hurdle. If we want the AI of tomorrow to be not only robust but also fair, we must create a technical and regulatory infrastructure that makes the diverse data needed to train AI algorithms available.
Healthcare is being transformed by a growing number of data sources that are continuously collected, transmitted, and fed to artificial intelligence systems. For new technologies to be accurate, they must be inclusive and reflect the needs of different populations. Addressing the complex challenges of AI bias will require collaboration between data scientists, healthcare providers, consumers, and regulators.
Data bias, information gaps, and a lack of data standards, common metrics, and interoperable structures represent the biggest threats to a transition to equitable AI. Incorporating open science principles into AI development and assessment tools can strengthen the integration of AI in medicine and open up space for different voices to participate in its use in medicine.
Postindustria offers a wide range of ML AI services and solutions. Leave your contact details in the form, and we’ll respond to discuss your custom solution.