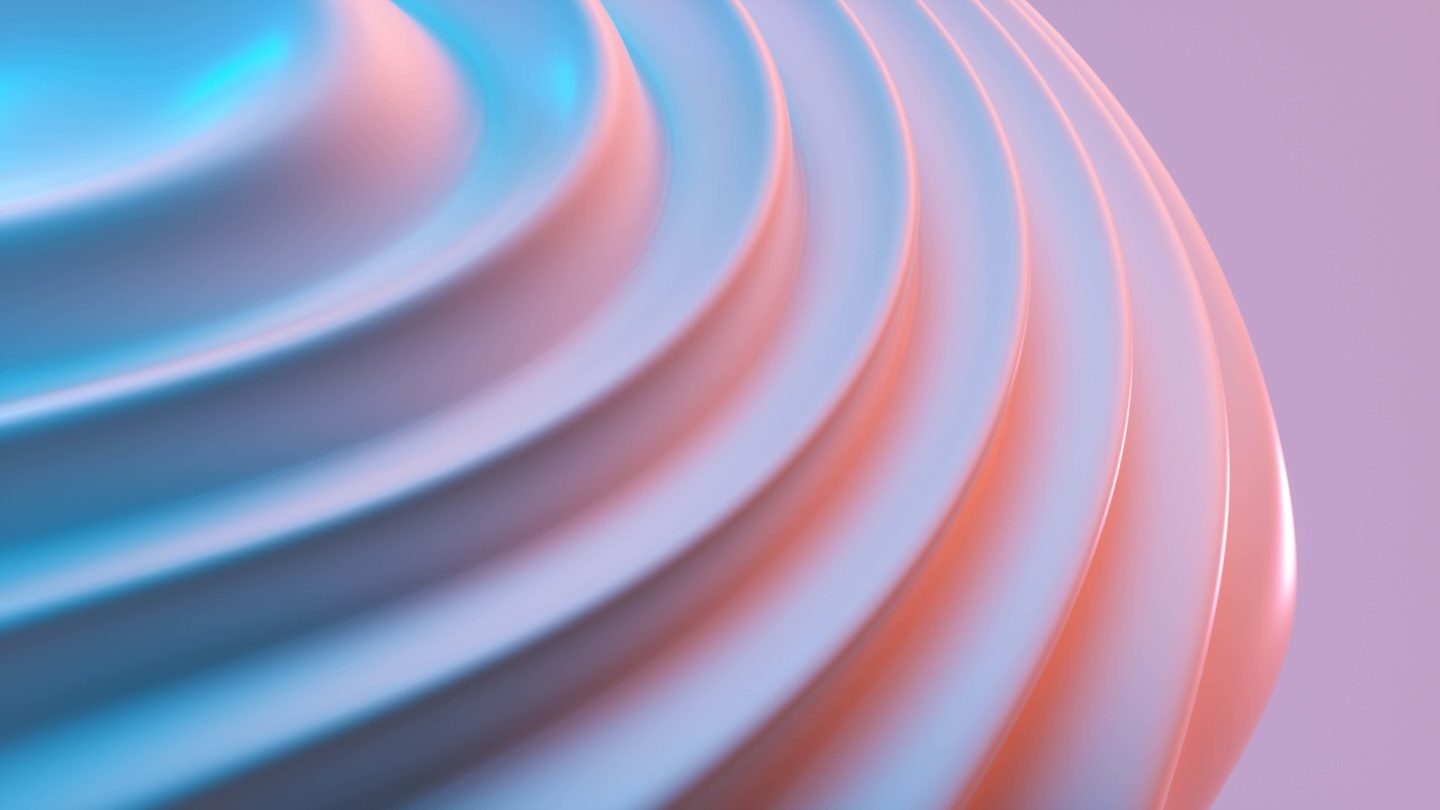
- Artificial Intelligence
Using AI Technology for Fashion Trends Prediction and Demand Forecasting
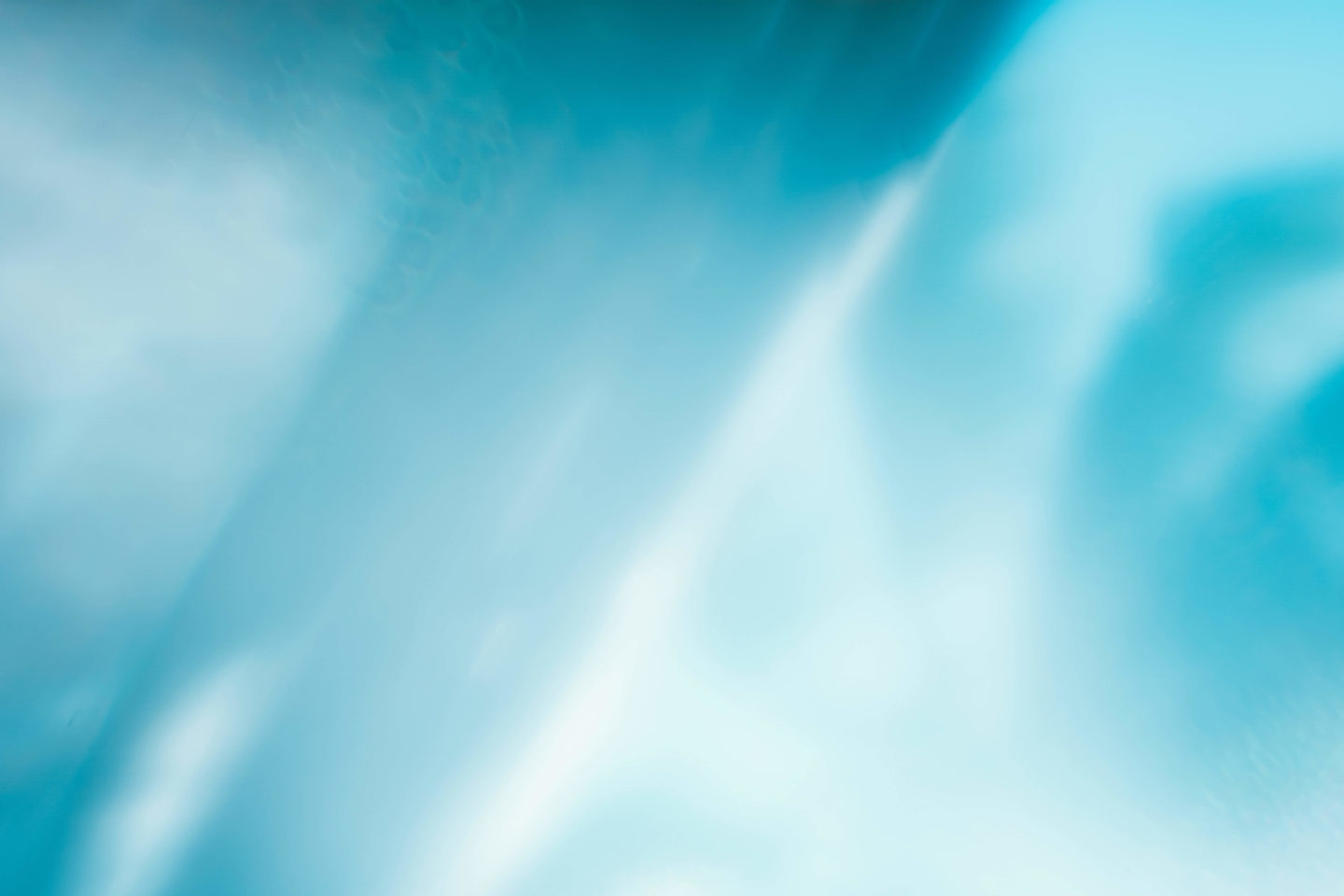
- Artificial Intelligence
AutoML Current Uses and Approaches
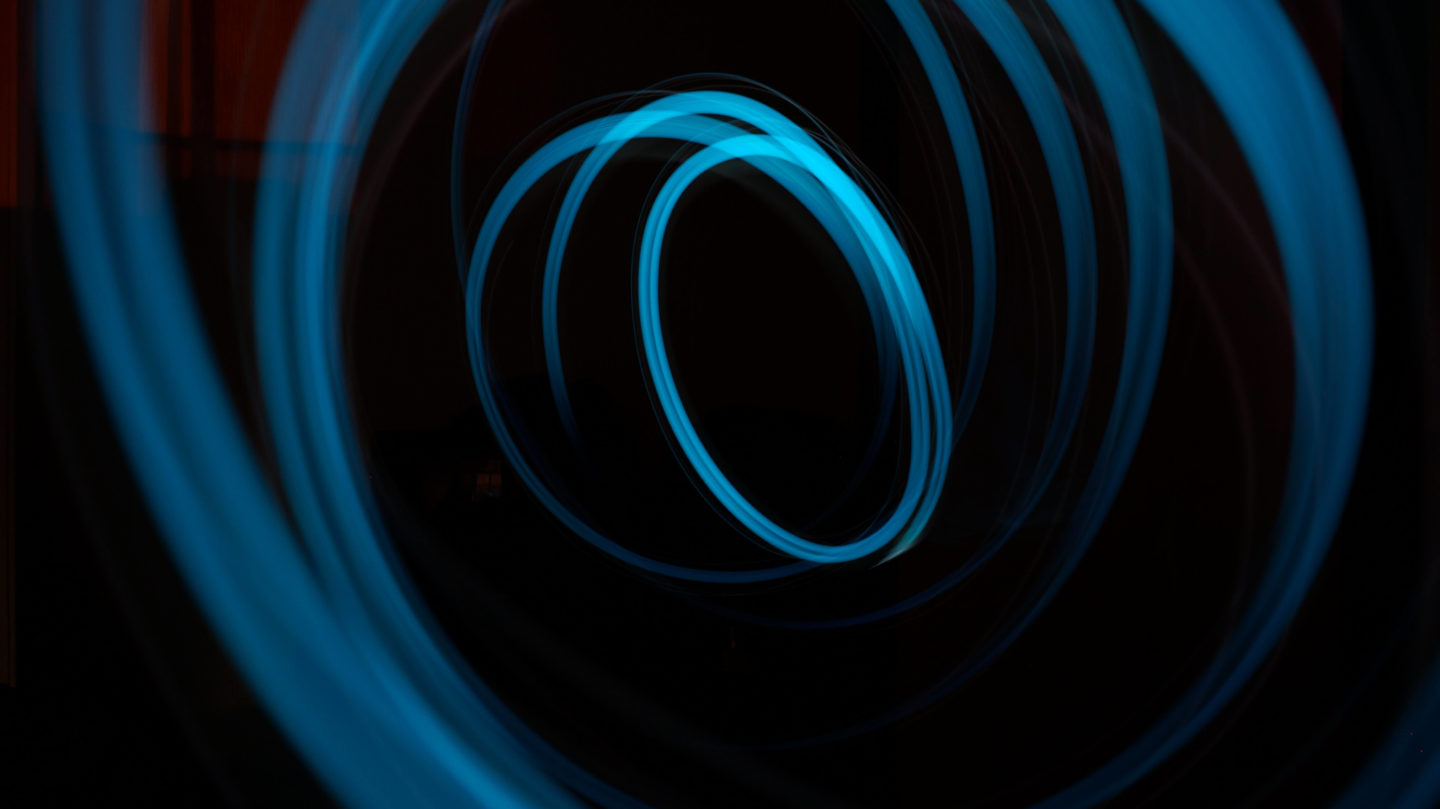
- Artificial Intelligence
According to McKinsey, the global market for AI-based services will reach $130 billion by 2025. And machine learning(ML) will be the dominant methodology. The rising demand for smart and automated solutions continues to drive market expansion.
As for manufacturing, adopting AI-powered technologies is a necessity for Industry 4.0. In fact, 64% of manufacturers already rely on AI in their day-to-day operations. And the area where AI is currently deployed the most is quality control. So why is AI for quality control so popular in manufacturing?
The reason is simple. AI-powered solutions help manufacturing companies enhance yield: according to IBM’s research, they can drive up to 30% improvement. Impressive? Read further to find out how your company can benefit from AI for quality control.
You may be wondering: is there a way to improve manufacturing yield without simply driving up costs? There is, and it involves reducing yield losses.
Yield losses are defective products that have to be reworked or disposed of. It’s the opposite of “yield,” which refers to the number of non-defective items. Reducing yield loss means ensuring that most products meet the quality standards. The main question here is how. Take a look at the steps below:
Previously, production engineers performed all these steps manually. But advanced quality-testing AI solutions perform them better. Linking process control data with quality control and yield data to identify yield loss is much more efficient than manual quality control.
Without a doubt, quality control is very complicated. Here are some of the challenges associated with the traditional manual approach to quality control.
By contrast, an AI-powered engine can analyze common defects and even classify manufacturing failure patterns. It identifies vulnerable aspects of production and forecasts failure. To put it simply, with respect to quality control, AI in manufacturing can outperform humans.
Thanks to machine learning, an AI-powered solution can be trained to classify data according to a rule-based system and then identify failures and defects early on. But how do AI and advanced ML algorithms for quality control work? Smart cameras and sensors gather images and data vital for quality inspection and ensure complete visibility of the production line.
Now, let’s have a closer look at the benefits of AI for quality control.
Thanks to computer vision and deep learning, AI-powered systems can quickly spot a problem in any part of the production line monitored by cameras or sensors. In fact, AI-based solutions can increase defect detection rates by up to 90%, compared to human inspection rates. This lets engineers spend their precious time addressing the issues, rather than manually inspecting for defects.
An AI-powered solution not only detects defects but also finds their root cause. If multiple failures occur, AI tracks their sequence. Even better, AI-driven root-cause analysis helps avoid manufacturing defects in the future. It reveals what parts of the value chain need changes, allowing engineers to continuously improve yield and reduce manufacturing defects.
AI quality control in manufacturing can determine optimal operating conditions. By analyzing various production data, it can automatically determine the key variables for such conditions. Thus, defects and product waste are minimized.
You can easily deploy one AI-powered system to different production sites. This means an engineer can quickly relay information about an emerging issue in one factory to another factory. An AI system can even integrate data from different production sites to improve predictions. Also, an advanced AI-driven solution can integrate with a company’s existing analysis tools and technologies.
An AI engine can perform quality testing faster than humans. It can fully automate complex tasks. Automated quality testing requires fewer person-hours than manual quality testing.
As you can see, AI-powered quality testing solutions have a lot to offer. Next, let’s examine how some manufacturing companies reaped the benefits we just described.
3B-Fibreglass is a developer and supplier of glass-fiber products and technologies for reinforcing thermoplastic and thermoset polymers. Their clients come from the automotive and wind-power industries. The manufacturing process consists of two phases. In the first, silica sand, limestone, and kaolin clay are heated up and melted to create glass. In the second phase, the molten glass passes from the furnace through the hole bushings to make fine filaments.
The main problem at 3B-Fiberglass was that the glass fiber kept breaking when pushed through the holes. The company used AI to gain insights into the root cause of the breakage. They implemented computer vision to monitor the flow of fibers and analyzed the data. At first, the AI learned to identify the break and revealed that it occurred on a particular bushing. Eventually, it could predict a break 75 seconds in advance. An AI-powered root-cause analysis found common patterns in all the breaks.
According to McKinsey, the highest overhead cost in the semiconductor industry is manufacturing. Semiconductor chip production cycles are long and have multiple steps. For instance, it usually takes several weeks or months to go from processing a silicon wafer to the final product. Various quality testing processes take place at each step along the way. Defects interrupt and delay production. Failure can occur at any stage of the production process, and data about defects isn’t always linked across different production phases.
AI for quality control identifies the root cause of yield losses and helps optimize chip design. Samsung, Google, and Nvidia are just a few companies using ML to improve chip manufacturing.
Although manufacturing companies can use AI systems throughout the whole value chain, it’s perhaps most helpful for quality control. AI defect detection outperforms manual testing processes. It brings multiple benefits, such as production optimization and root cause analysis. Most importantly, AI-driven quality control in manufacturing enhances yield. Thanks to deep learning algorithms, AI models continuously learn and show even better results over time.
Postindustria offers a full range of services and delivers solutions based on machine learning. Leave your contact information in the form above, and we’ll reach out to discuss your project.
Thank you for reaching out,
User!
Make sure to check for details.