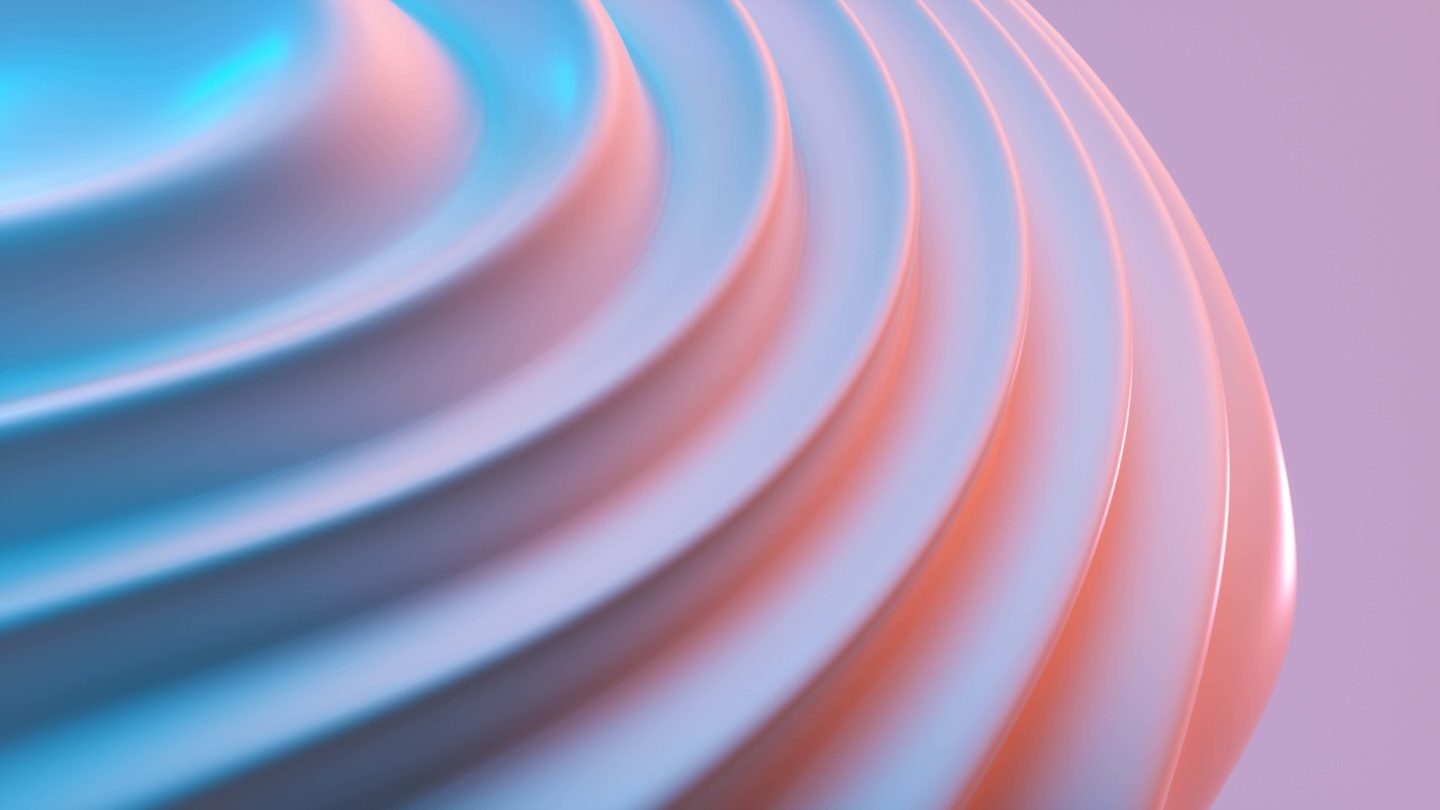
- Artificial Intelligence
Using AI Technology for Fashion Trends Prediction and Demand Forecasting
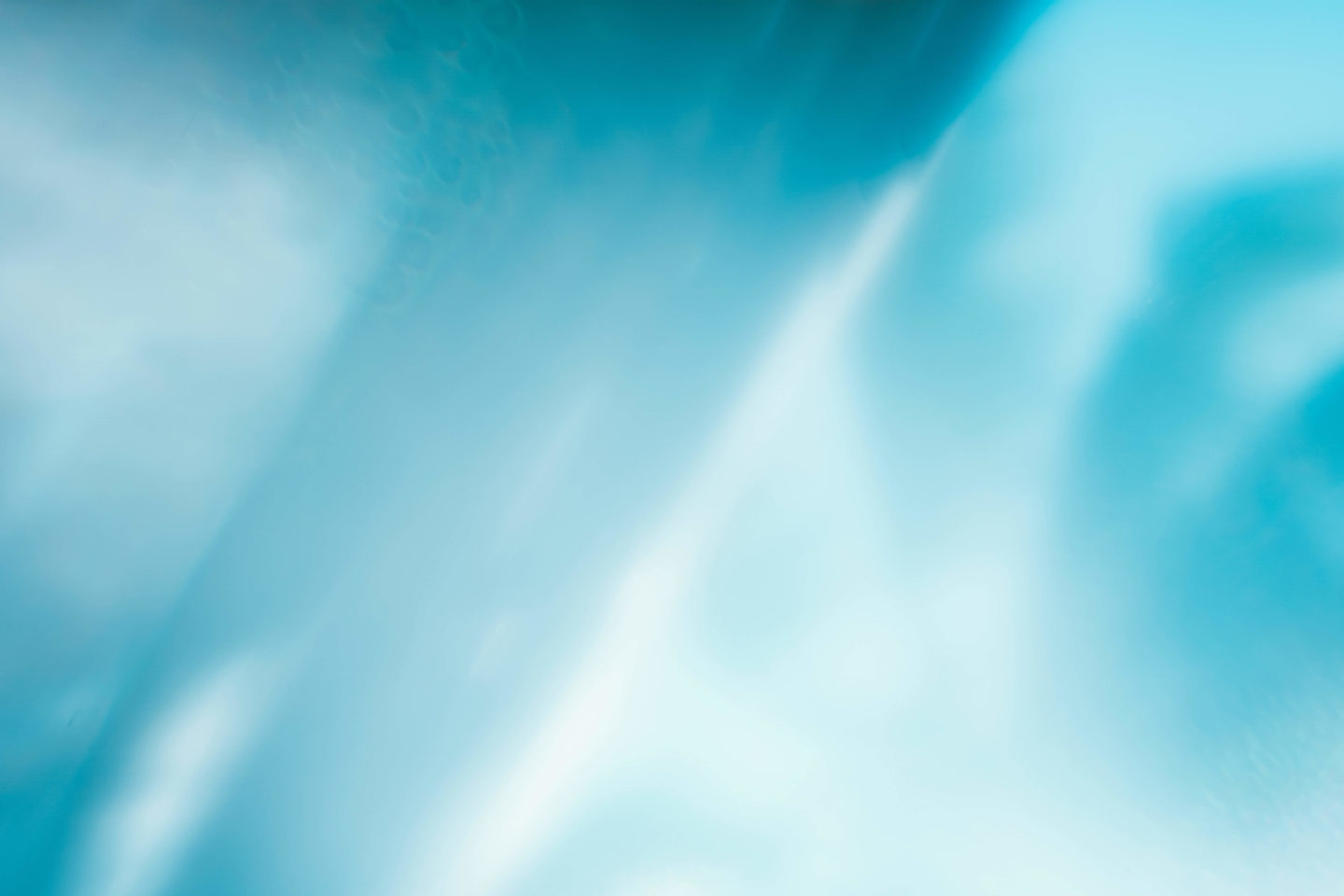
- Artificial Intelligence
AutoML Current Uses and Approaches
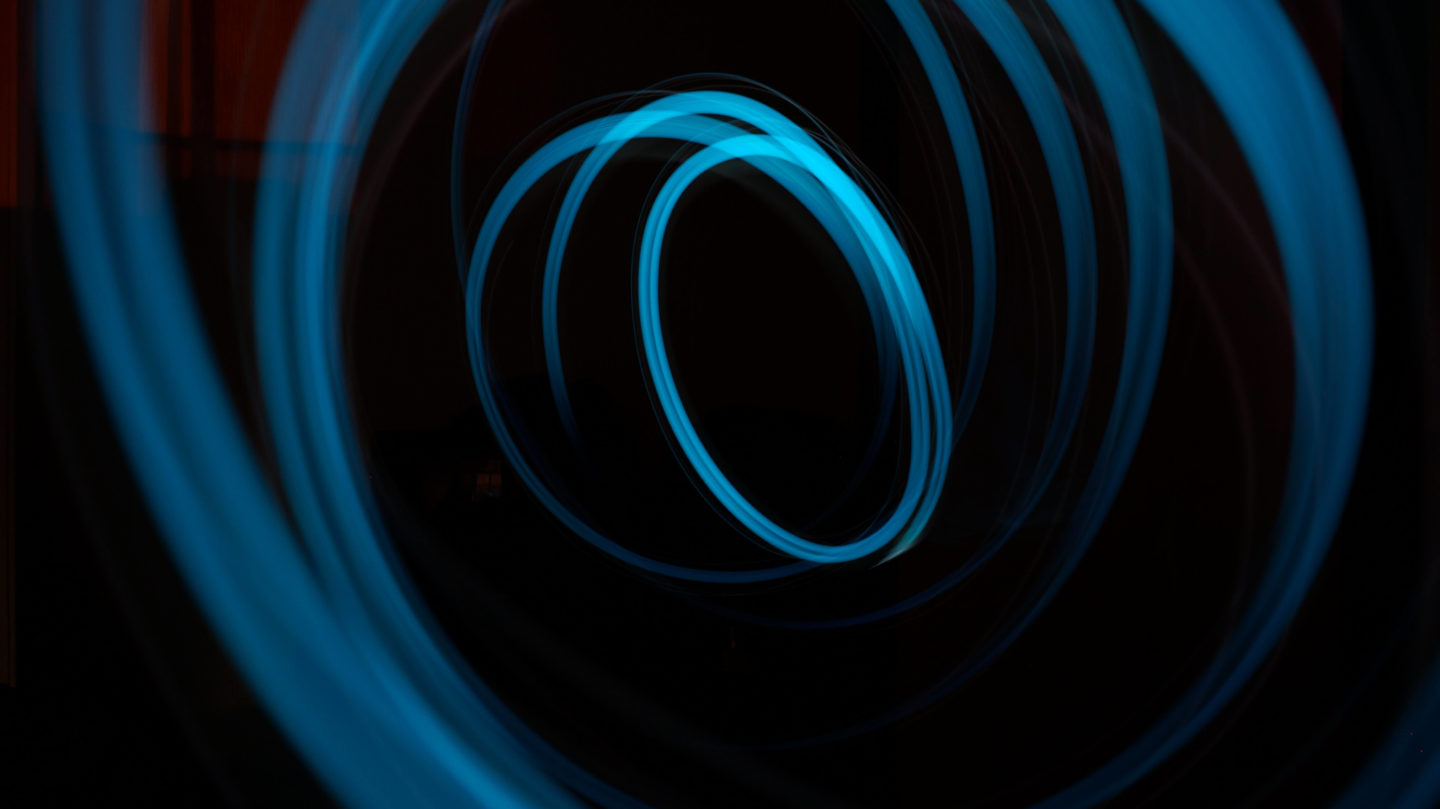
- Artificial Intelligence
Years ago, British mathematician and Tesco marketing executive, Clive Humby, coined a phrase: “Data is the new oil.” In 2022, this expression is as relevant as ever.
According to Statista’s predictions, the worldwide volume of data will reach 120 zettabytes by 2023. A zettabyte is enough to store 60 billion video games or 30 billion 4K movies. In 2020, this number was slightly higher than 64 zettabytes.
From a healthcare perspective, large volumes of data can bring great value. Analysis of the enormous amount of data stored in electronic health records (EHR) or electronic medical records (EMR) can inform clinical research and clinical decisions in numerous ways.
However, when a healthcare project relies on analyzing EMRs, specialists frequently face a challenge — interpreting clinical text. Information about patient conditions is often indecipherable by non-clinicians and requires medical experts to clarify. This presents a roadblock to developing innovative solutions for healthcare at scale.
The good news is that there is a solution: natural language processing (NLP). Applying NLP in healthcare research automates the extraction of meaningful data from EHRs to speed up clinical workflows, enforce consistency, and produce better patient outcomes.
So how is NLP used in healthcare and how can medtech startups leverage its potential to benefit patients?
NLP refers to machine learning (ML) algorithms that process and analyze human language or text, without human intervention.
Popular tools and devices, like Google Translate, Apple’s Siri, and automatically generated text suggestions while writing an email all have NLP technology at their core.
The application of NLP in the healthcare industry serves two primary functions:
The first area of application involves developing ML models based on a principle similar to AI-enabled voice assistants. The goal here is to teach a computer model to recognize medical terms in clinicians’ speech, spot patterns, and make informed predictions about patient conditions or suggest treatment plans.
The second focus consists of algorithms to isolate meaningful data from unstructured medical records. This application helps decision makers find the information they need to make informed care or business decisions quickly.
The two most important NLP techniques used in the healthcare industry are:
ML models that use statistical techniques to learn from training data input over time; and
ML engineers embedding NLP into healthcare projects might rely on the following approaches:
According to an MIT analysis, ML models can work only with conditions and diseases that occur regularly in at least 1% of the population, as this guarantees that the disease is not a random occurrence. With a 1% incidence rate, it’s possible to have a sample with enough information to train a model to discern insights.
Although support vector machine (SVM) learning is no longer state-of-the-art in ML, it is simple to use and comprehend. Its uses include entity recognition in text and it can be trained to select drugs for patient treatment and diagnose diseases.
Classification trees are supervised ML models that separate data into distinct classes. The results are discrete values and probabilities of their occurrence.
Bayesian networks are built on algorithms that predict diseases based on symptom occurrence or co-occurrence of a cluster of symptoms or features obtained from large datasets.
Artificial neural networks are complex models that imitate the human brain and it’s outcomes have the highest precision of all the algorithms. They’re implemented over a character sequence in a textual data file, accounting for misspellings and compound medical terms. Neural networks constantly feed and improve themselves and learn from previously tested models.
The answer to this question is yes and no. Despite Moore’s law, NLP technology is slow to develop and it’s premature to speak about its clinical applications at scale.
However, in the area of computer-assisted medical coding, tech giants have made significant progress lately.
In May 2020, IBM reported the availability of its Watson Annotator for Clinical Data on IBM Cloud. This NLP-driven tool analyzes text to extract and code metadata from unstructured text, including contextual data for diseases, lab values, medications, and procedures.
Another tool, Isabel, uses sophisticated NLP software to extract key features from medical records, including vital signs, lab test results and demographic factors, and match them to diseases.
Medical speech recognition and dictation solutions from Phillips and Nuance’s Dragon Ambient eXperiencce (DAX) help doctors instantly create medical documents and make them available for central administration and healthcare information systems. These user-friendly solutions for recording dictation and patient interactions require no extra time to produce reports, which are instantly saved to the cloud.
3M’s M*Modal tool is an NLP-enabled cloud-hosted voice assistant that lets clinicians dictate their observations about patient’s conditions from anywhere using any device, allowing hospitals and clinicians to enrich the content of EHRs.
As few as 3% of patients with a given condition participate in clinical trials, solutions like IQVIA’s Decentralized Clinical Trials (DCT) that increase participation rates, maximize retention, and improve data quality, are essential.
Navify is an example of decision support systems that assist doctors by looking not only at historical patient data, but also at the latest outcomes of related drug trials and research guidelines. They then propose treatments options and doctors can select and offer the optimal patient treatment. This is especially useful for cancer treatments because so many trials and factors inform oncology treatments.
Taking into account the advances described above, let’s summarize some key benefits that the application of NLP in healthcare projects can yield:
Of overarching importance, applying NLP in healthcare at scale has the potential to benefit all stakeholders — patients, healthcare providers, and clinical operations managers.
Use of NLP is likely to be a trend to watch in the medtech industry in 2022 and beyond. Advances in NLP will accelerate development of its clinical applications, enhance patient care, and optimize clinical practices.
If you’re looking for a reliable NLP service provider, choose Postindustria. Our team of AI specialists led by PhD-level engineering managers has extensive experience integrating ML-enabled technologies into product workflows.
We’ve successfully delivered US-based medtech projects and can help deploy or upgrade an NLP-driven ML model for your custom solution. Check out our Mahmee and Mindbloom healthcare projects and book a strategy session with our experts to discuss how to level up your game.
Thank you for reaching out,
User!
Make sure to check for details.