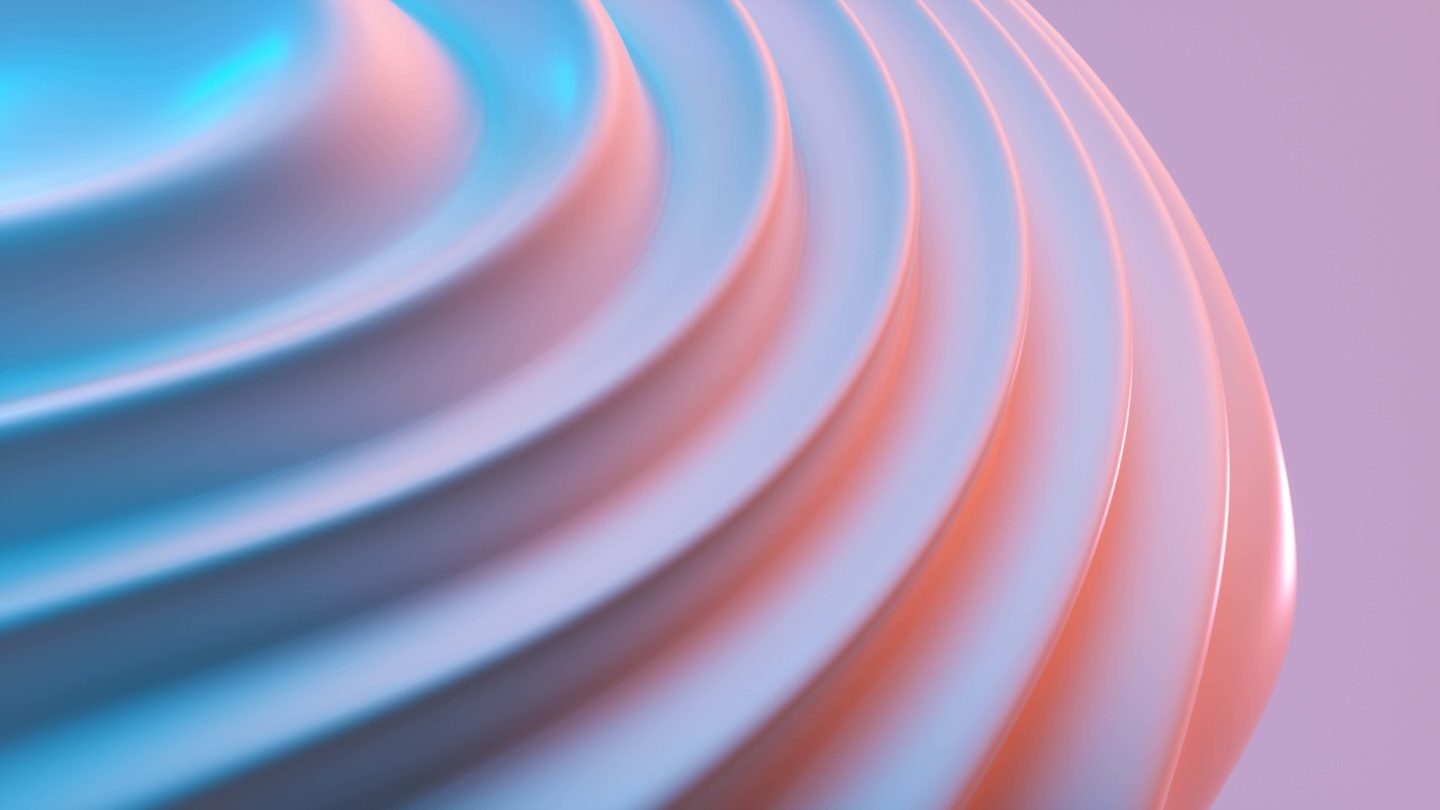
- Artificial Intelligence
Using AI Technology for Fashion Trends Prediction and Demand Forecasting
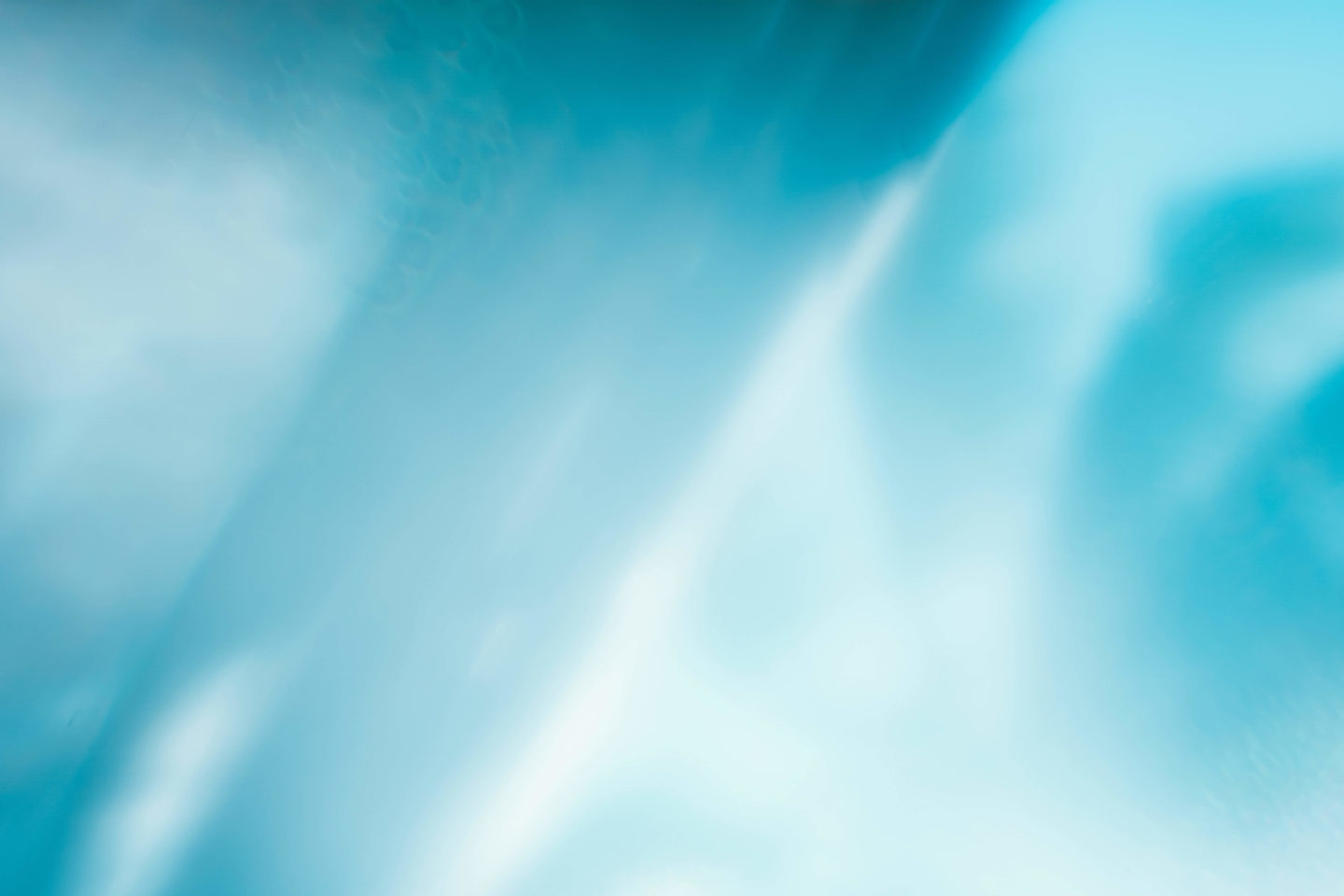
- Artificial Intelligence
AutoML Current Uses and Approaches
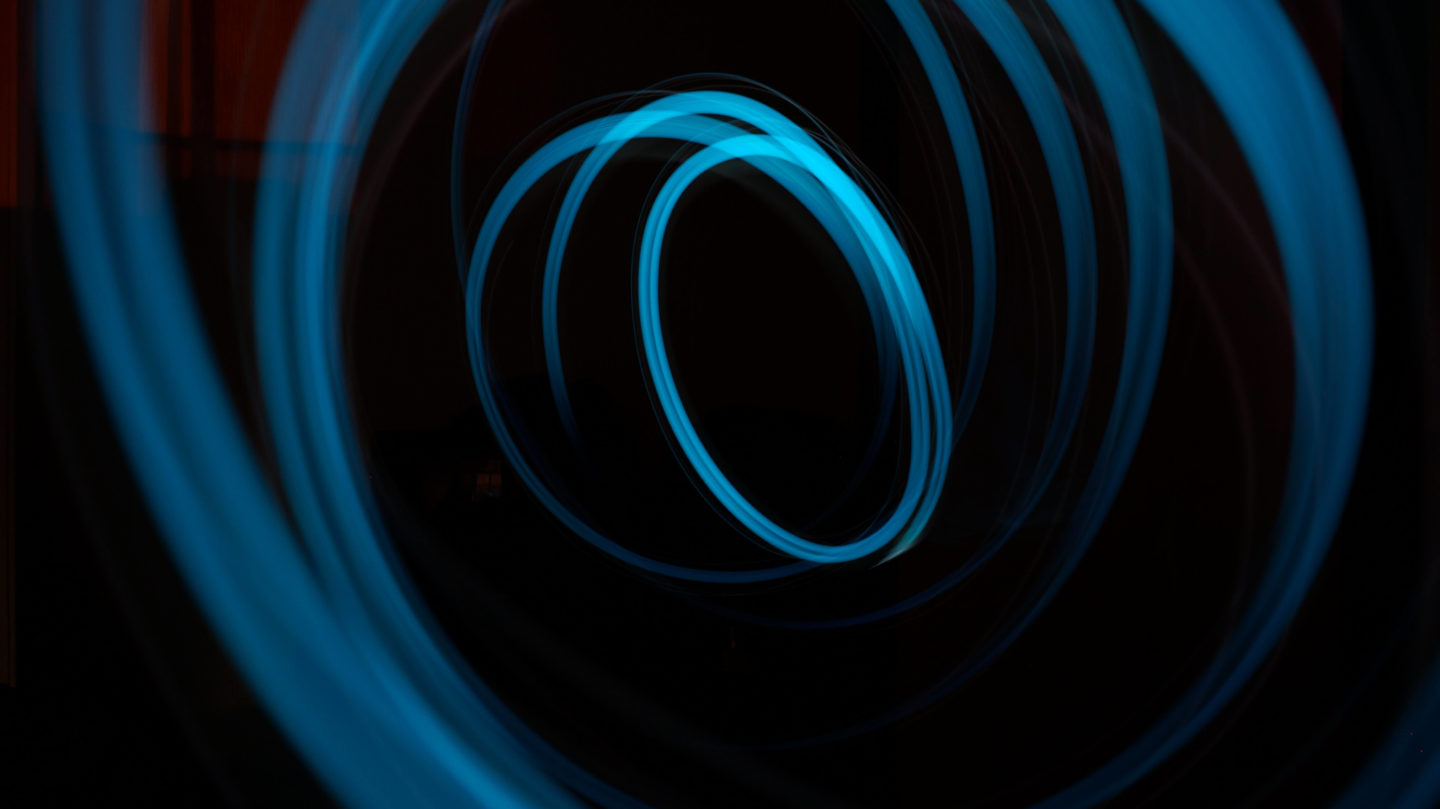
- Artificial Intelligence
Artificial Intelligence (AI) shows enormous promise in diagnostics, symptom prediction, and risk identification. The global market for algorithm-based healthcare solutions could grow from $6.7 billion in 2020 to $120.2 billion by 2028. But despite the aggressive growth of AI in healthcare, challenges hinder its adoption in clinics and diagnostic centers.
This article explores the potential uses of AI in medicine and the barriers to its deployment in clinical practice. Read on to also learn how AI algorithms can help your company today.
AI is a set of techniques, tools, and algorithms that power systems with the goal of simulating human cognition. When someone mentions AI in healthcare, chances are they’re referring to a subcategory of AI called machine learning (ML). ML is a branch of AI that uses external data to learn new tasks without being explicitly programmed to learn them.
AI has made major leaps in usage since 2016. According to the 2021 AI100 report, it’s now undergoing active testing in health centers for diagnosis, symptom prediction, and research, including drug discovery. Some of its most promising use cases include:
But how far is the current state of AI from realizing this potential? Let’s look at the main challenges for its adoption to find out.
Despite impressive possibilities and an array of overly optimistic studies, real deployment of AI-enabled solutions in clinical practice is scarce. Let’s explore the biggest technical, methodological, and privacy challenges of using AI in healthcare.
Clinicians need high-quality datasets to technically and clinically validate AI models. However, obtaining patient records and images to test algorithms is difficult because medical data is fragmented across different EHRs and software platforms.
The medical data from one organization can be incompatible with other platforms due to interoperability issues — a significant challenge for AI in healthcare. According to a 2019 HIMSS Media research report, only 36% of systems can recognize terminology, medical symbols, and coding values automatically. The healthcare industry must focus on standardizing medical data to make more data available for testing AI applications.
The metrics used to measure the performance of AI models don’t always generalize to clinical applications. This is known as the AI chasm — the gap between the technical accuracy of AI tests and clinical efficacy in the real world.
According to a 2019 report titled “Making ML Models Clinically Useful,” there’s little evidence that AI models for diagnosis and health prediction can improve patient outcomes. AI researchers use several indicators to measure the performance of AI tools, but those indicators don’t necessarily represent clinical effectiveness.
Besides, these metrics vary across studies because of insufficient metrics standardization. For instance, comparing the effectiveness of AI models for COVID-19 is nearly impossible because there’s no standardization.
Clinicians and developers should first and foremost jointly test how AI algorithms improve patient care. To achieve that, they can use decision curve analysis to evaluate models based on accuracy and clinical applicability. The clinics will have to do additional research to determine the threshold probability for different categories of patients. After all, the AI model might be inaccurate for some of them.
Studies of AI in healthcare suffer from a lack of standardized methodology, prospective studies, and peer-reviewed evidence.
The majority of research has been retrospective, meaning it relied solely on historical medical data of diagnosed patients. But to fully understand the true utility of AI diagnosis and treatment software in real-world settings, clinicians must study current patients over time (prospective research).
Doctors should follow their patients’ health over time to test the reliability of research. Clinicians can combine physical examinations with telehealth visits and remote monitoring tools (sensors and trackers) to study patients continuously.
AI studies rarely go through peer reviews and randomized controlled trials (RCTs), impairing their validity. A 2019 study published by The Lancet reported on an AI platform that performed well during specific prospective studies, but was less accurate than senior clinicians in an RCT.
A 2021 report in Nature Machine Intelligence identified methodological flaws and biases in studies of AI algorithms used for COVID-19 detection. The systematic review of 62 studies of COVID-19 ML models concluded that none were ready for clinical use.
In 2019, the Korean Journal of Radiology reported that only 6% of published papers on AI systems for medical image diagnostic analysis had any external validation. Plus, nearly all studies failed to validate their real-world clinical performance.
AI-powered systems with ML models can show unreliable results if the data used to train them is biased.
Training models receive input data from non-stationary environments, like clinics serving many populations and with evolving operational practices. Shifts in demographics and clinical practice can create bias when an algorithm is trained with data collected under constantly changing conditions.
Bias also comes from patient demographics, such as race, gender, and socio-economic factors. For instance, AI trained on data from academic centers in big cities will provide less accurate predictions for rural patients. Even worse, the model can exacerbate existing inequalities in the healthcare system instead of reflecting objective reality.
Organizations can reduce bias by training the algorithms with more diverse data. Sometimes it’s possible to augment the base data with external samples to add balance, but this method doesn’t work with all AI solutions and data types.
Thank you for reaching out,
User!
Make sure to check for details.
AI-powered solutions with machine learning algorithms use all available input data to improve their performance. Sometimes, AI systems exploit variables researchers didn’t anticipate it would use, which can confound results.
For example, some algorithms classified skin lesions as malignant on dermoscopic images because they contained surgical skin markings or rulers. Another AI system accurately identified patients at high risk for pneumonia based on X-ray images from Mount Sinai Hospital, but performed significantly worse with images from other facilities. As it turned out, the system could have identified high-risk patients in the original hospital by recognizing which machines scanned intensive care patients, a confound researchers might not anticipate.
Data scientists must examine data carefully before feeding it to an AI module. A popular technique is to use exploratory data analysis (EDA) with feature engineering (FE). EDA summarizes the data sets’ main characteristics to simplify pattern and anomaly detection, and FE automatically determines optimal features for the model.
Understanding how AI determines diagnoses or predictions is paramount for healthcare, especially for clinical decision support systems (CDSSs). This includes understanding both the general inputs and the features used for individual predictions.
Conventional AI solutions, such as artificial neural networks (ANN), are black-box models. This means you can’t know how the system comes to its conclusions.
Transparency and explainability are often cited as primary challenges and disadvantages of using AI in healthcare in its current form. In a 2020 article titled “Three Ghosts of Medical AI,” specialists concluded that the inability to understand how a system arrives at recommendations restricts the iterative knowledge-discovery processes.
Clinics must follow laws regarding protected health information in medical records, namely HIPAA and FDA regulations. However, following these regulations doesn’t automatically guarantee compliance and data privacy.
ML-enabled solutions can learn to detect patterns or biomarkers and process them as medical information. For example, an algorithm could identify a patient’s Parkinson’s disease based on the hand trembling while using a computer mouse. The patient might consider this a privacy violation, especially if the computer he was using was linked to third parties (employer or insurance company) or if the patient didn’t ask for a diagnosis.
Clinics must seek patients’ informed consent in advance to avoid privacy breaches. This means the physicians should state if they don’t know how their black-box modeling algorithms produce suggestions based on initial data.
Notably, some types of AI systems don’t fall under FDA regulations (either because they don’t perform medical functions or because they’re developed and deployed in-house). The agency also doesn’t have precise requirements for transparency and explainability—other important challenges of implementing AI in healthcare.
Despite technical, ethical, and privacy challenges, AI in healthcare is common today. Clinics, research facilities, and diagnostic centers rely on features such as:
The existing AI-driven solutions can improve productivity, save time, and ultimately, help doctors provide better patient care.
Sophisticated AI models aren’t yet ready for mass deployment because of data biases, transparency issues, and privacy protection concerns, but all of these challenges are solvable. More importantly, the potential benefits of AI-enabled systems far outweigh the effort needed to perfect them.
AI presents exciting opportunities to improve healthcare with diagnostics, early symptom predictions, and drug development. The technology can help doctors see a complete picture and consider alternative diagnoses and treatment options. On top of that, current AI- and ML-based systems are already helping companies improve their workflow.
Postindustria can empower you with advanced AI development solutions, including natural language processing, big data analytics, and robotic process automation. We can also create an AI system from scratch according to your technical requirements and specifications. Reach out to our team to see how we can bring your concept to life!