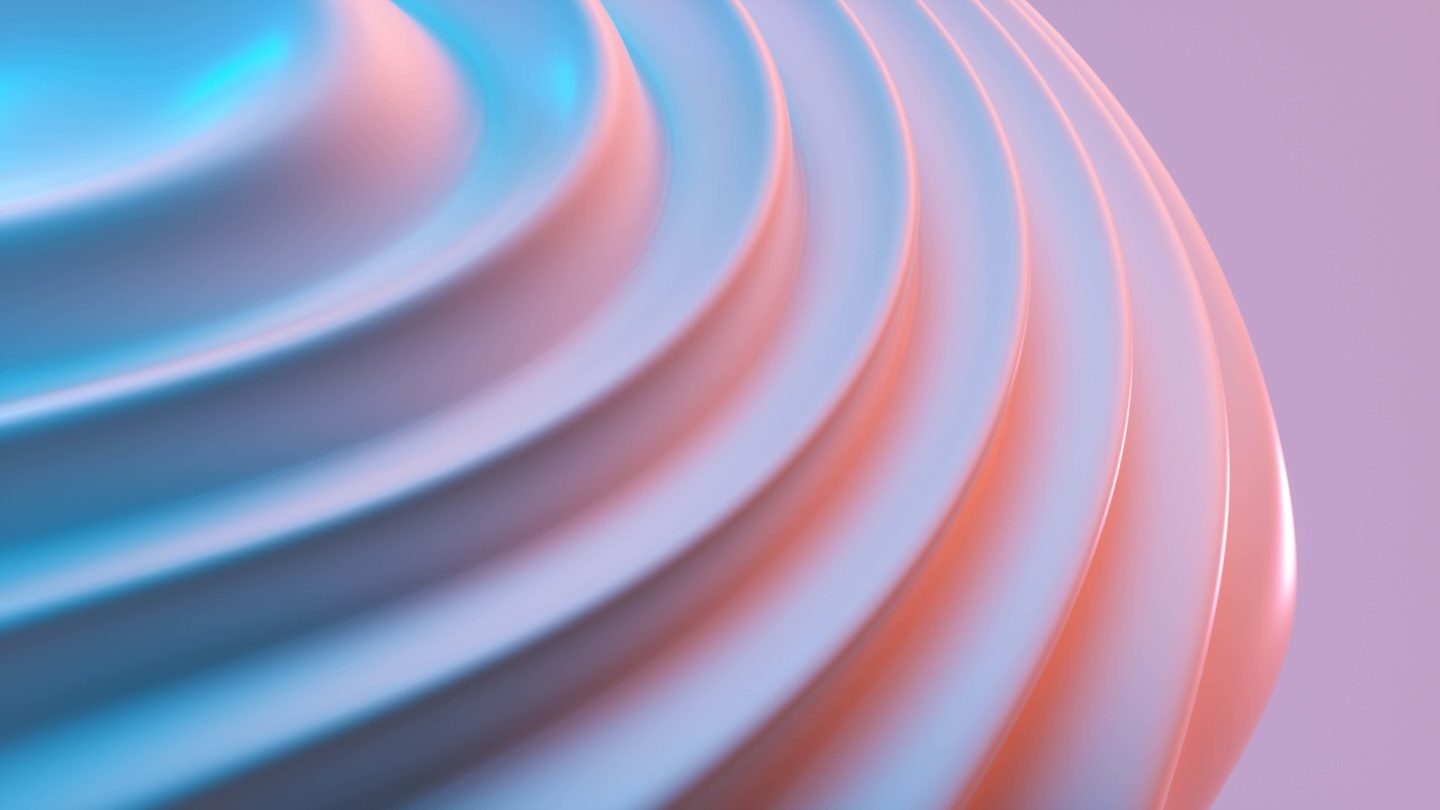
- Artificial Intelligence
Using AI Technology for Fashion Trends Prediction and Demand Forecasting
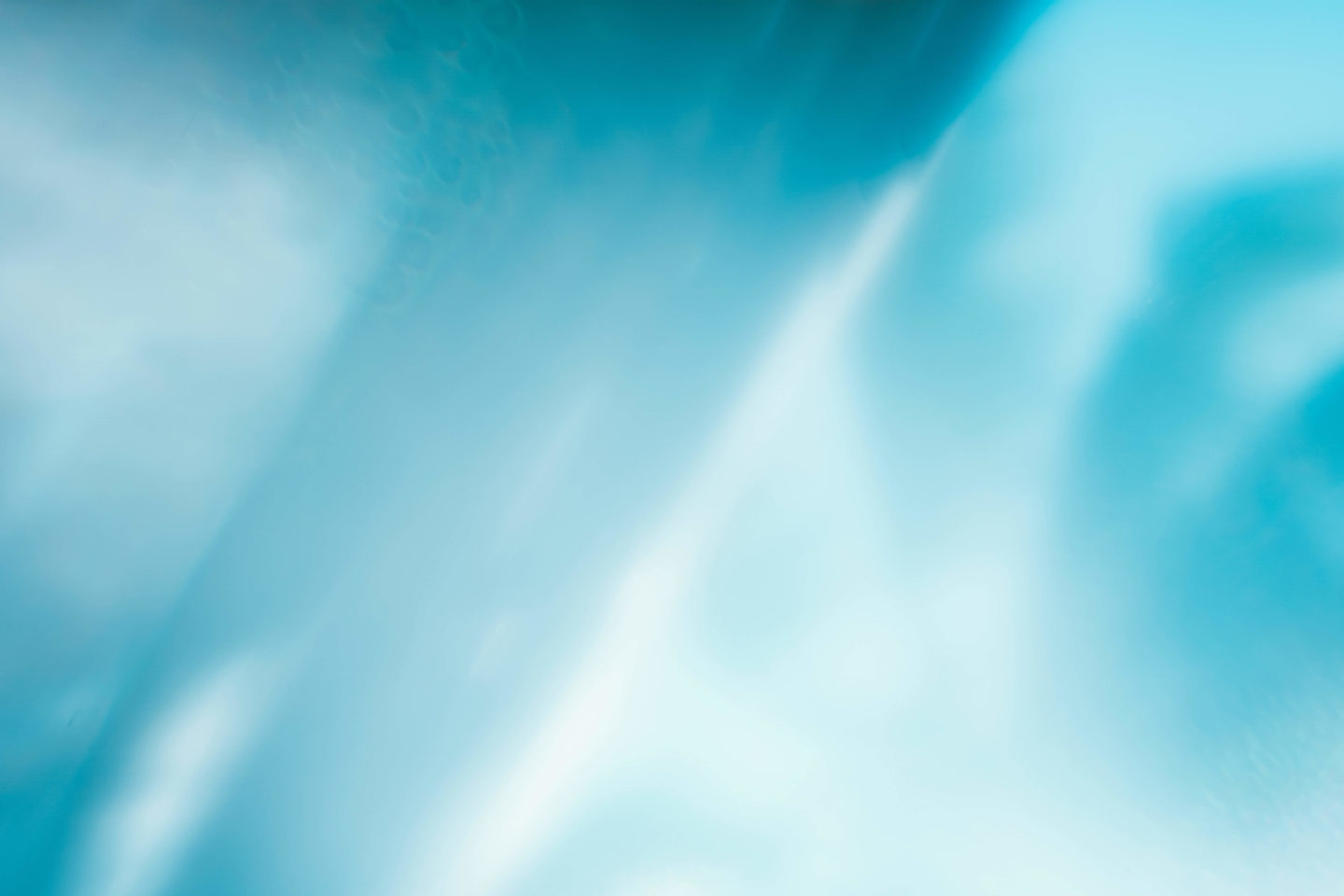
- Artificial Intelligence
AutoML Current Uses and Approaches
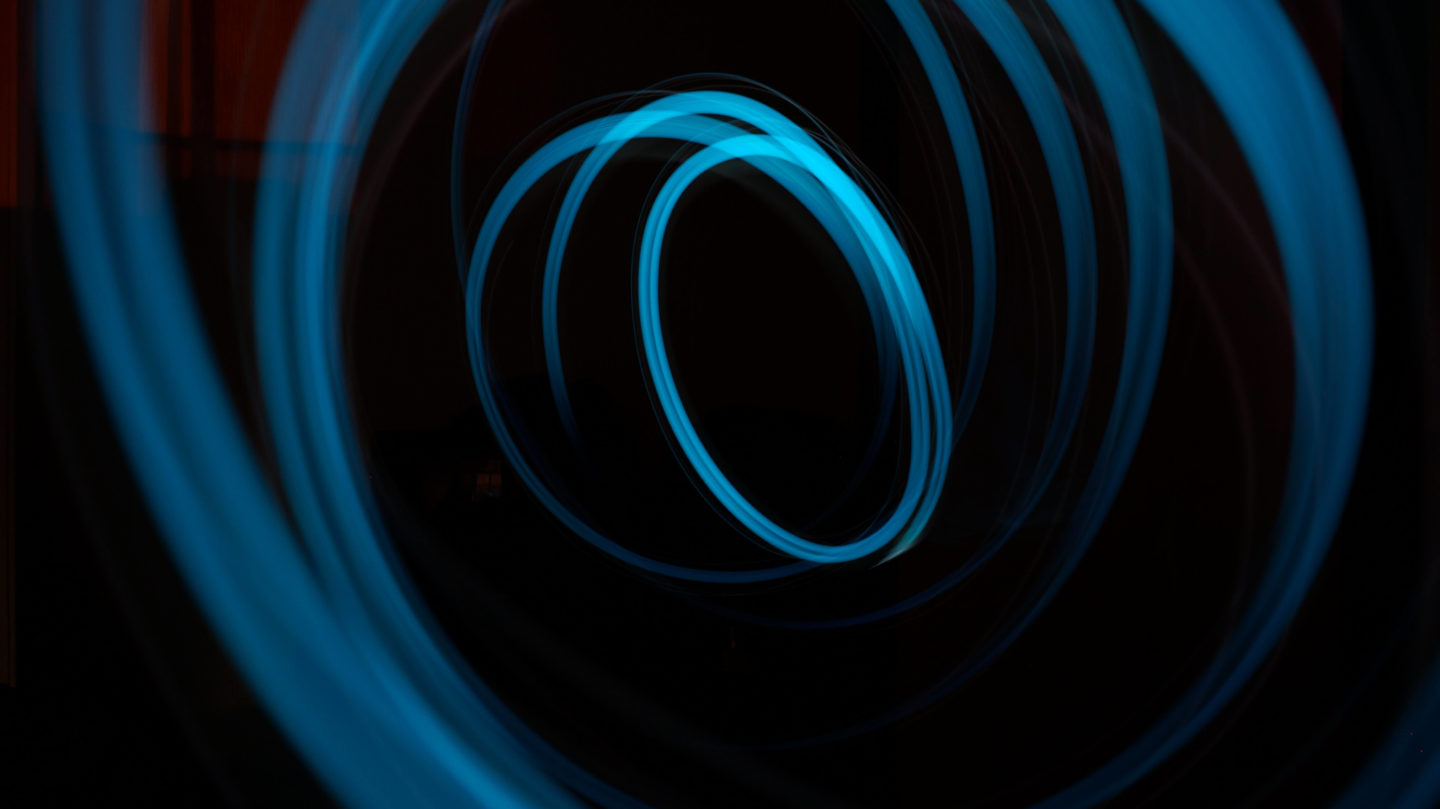
- Artificial Intelligence
Artificial intelligence (AI) is a broad term with almost unlimited interpretations and just as many misconceptions. AI talks are full of confusion and misinformation because we use the word “AI” to refer to so many things.
A study published in 2016 suggested that surveyed AI experts predicted with a probability of over 50% that AI systems will reach overall human ability by 2040-2050. Stuart Russell, the co-author of a widely used textbook on AI, predicts that superintelligent AI is likely to emerge within the next generation’s lifetime. While Sam Altman, CEO of AI company OpenAI, predicts that in the coming decades, computer programs will do almost everything, including making new scientific discoveries that will expand our concept of “everything.”
There are a lot of such examples when well-known researchers or developers in the field of AI made forecasts suggesting that AI was rapidly gaining ground, generating even more myths and fears around AI. However, despite all the fuss, AI hasn’t reached its peak stage and indeed is still far from it.
People’s views differ greatly depending on which gate served as their introduction to the world of AI. In this post, I’ve collected the misconceptions about AI that our company encounters the most, even from tech specialists.
While AI will radically change how work is done and who does it, the technology’s greater impact will be to complement and enhance human capabilities rather than replace them.
Today, experts train machine learning algorithms to do the job they are designed to do. People are teaching machine translation applications to process idiomatic expressions, teaching medical applications to detect diseases. AI increasingly draws conclusions through non-transparent processes, which has legal ramifications. For example, the new EU General Data Protection Regulation (GDPR) gives consumers the right to receive an explanation for any algorithm-based decision, such as offering a rate on a credit card or mortgage. This is one area where AI will boost employment, with experts estimating that companies will need to create around 75,000 new jobs to meet GDPR requirements.
The field of medicine also demonstrates that the combined efforts of scientists and machines have produced a greater effect than either of them could achieve separately. A team of pathologists at Harvard has developed a method to detect breast cancer cells. It was able to accurately identify cancer cells about 92% of the time. While the pathologists’ readings were 96% accurate. An even bigger breakthrough was that combining the analysis of the pathologist with an automated method of computer diagnostics improved the result to 99.5% accuracy. The collaborative work of machines and humans has led to a significant reduction in errors.
The self-driving vehicle industry also requires human experts. They are needed both in the tactical process of driving a vehicle and in the cognitive processes required to recognize common traffic signs and obstacles on the road. Thanks to its quick response and the ability to perfectly remember the rules of the road and comply with them, AI outperforms human drivers in standard road conditions. However, these advantages of AI are practically erased as soon as something happens that the AI has not been trained to deal with. When that happens, AI processing power must give way to human adaptability.
Perhaps the most popular and potentially dangerous misconception about AI is that it will put people out of work. Many people were concerned with the World Economic Forum (WEF) report estimating that by 2025, 85 million jobs may be displaced by a shift in labor division between humans and machines. However, in the coming years, this only means the disappearance of entry-level positions that involve routine tasks.
Thanks to advances in technology, some computers can perform business processes without human bias. Natural Language Processing (NLP) allows chatbots to understand speech and provide technical support to customers across industries, including food and retail. Human resources departments and finance companies use robotic process automation (RPA) to validate payroll systems, generate email reports, and manage expenses, among other tasks typically performed by employees.
However, the same WEF report also states that there will be 97 million new job openings as a result of this shift. As more computers are trained to perform the frequently repetitive tasks often assigned to entry-level employees, the more complex task-focused, competitively paid roles will spring up in their place. This means that young professionals can have a wider choice of interesting professions.
This statement has been discussed since the beginning of computing. But it has received a lot of attention in recent years as advances in machine learning methods have given us a more concrete understanding of what we can do with AI, what AI can do for us, and how much we still aren’t doing.
Victoria Krakovna, an Alphabet researcher, has compiled a list of examples of a “specification game”: the computer does what we tell it to do, but not what we want it to do. For example, the AI had the task of putting a red block on top of a blue block. To be more precise, the player must be rewarded for making the bottom face of the red block high above the floor. Instead of picking up the red block and putting it on top of the blue one, the AI just flipped the red block over. Thus, the goal was achieved (high bottom face of the red block).
A more complete specification of the desired result would also include that the top face of the red box should be higher than the bottom face and that the bottom face is aligned with the top face of the blue box.
Consider a more serious case of unpredictable AI behavior. Specifically, the development of expert systems to assist judges in the United States. The training dataset had an imbalance of more Black people being convicted, and therefore the neural network began to give preference to white people when forming a court opinion. To remedy the situation, the developers adjusted the datasets by removing all personal information that can be correlated with race: names, surnames, mentions of appearance. But this did not force the neural network to abandon rating by skin color: it learned to recognize race by a person’s address because, in the U.S., many African Americans live in neighboring communities.
Thus, in real life, AI does not always follow the scenario that the programmers assumed. This brings us to the concept of AI safety. AI safety can be broadly defined as the desire to ensure that AI is deployed in a way that does not harm humanity. AI behavior depends on what target function the creators prescribed for it. As the examples above show, it is not enough just to indicate the goal. You need to set an additional set of rules and conditions for the AI to interpret its task correctly.
Sometimes it seems that in the 21st century we face dangers from all sides. Unfortunately, we have a clearer understanding of the policies we need to adopt to address climate change than those we need for AI. However, today most modern applications are used to positively impact humanity. There are many AI applications that make our daily life more convenient and efficient.
Despite AI’s mind-blowing progress, this notion is incorrect. AI cannot understand or make sense of its environment, nor can it truly “learn” from its environment in the way humans can. For example, Siri or Alexa can make appointments, but they sometimes give chaotic answers when a conversation goes off track.
The most popular AI techniques are indeed called neural networks and they are inspired by the biological brain. However, it is important to note that despite the name “neural network”, such models are not physiological neural models because neither the model of a neuron nor the connection between neurons in AI neural networks is biologically plausible or realistic. This means that neither the connectivity structure of convolutional neural networks, feed-forward neural networks, nor other deep learning architectures are biologically realistic.
Cognitive AI can identify an image or analyze the meaning of a sentence, but it definitely needs human intervention. When Facebook tried to identify relevant news to present to users, the automated process failed to distinguish real news from fake. In fact, Russian hackers managed to post fake news without detection by the automated filters, so Facebook decided to hire a team of editors to monitor the News Tab. That’s just one example of security lagging behind the performance. There are also certain patterns developed to trick algorithms into misclassifying objects layered on images. Cognitive technologies are a great tool, but the human brain is still far superior.
To give machines common sense would require imbuing them with some basic concepts, perhaps innate knowledge that human infants have about space, time, cause and effect relationships, the nature of inanimate objects and other living beings, and the ability to draw analogies from previous experience. No one yet knows how to capture such knowledge or abilities in machines.
Technically it could be. An AI system can only be as good as its inputs. If you can clear your training dataset of conscious and unconscious assumptions about race, gender, or other ideological concepts, you can create an AI system that makes unbiased decisions based on the data. However, in the real world, we don’t expect AI to ever be 100% objective.
An interesting example is an Amazon recruiting tool, which showed bias against women. The company’s experimental hiring tool used AI to rate job candidates by giving them 1 to 5 stars. The project was purely based on reviewing applicants’ resumes so that recruiters don’t waste time on manual tasks. However, by 2015, Amazon realized that its new AI-based hiring system was unfairly evaluating candidates and exhibiting a bias against women.
The issue was that the company used historical data for the last 10 years to train its AI model. This data contained a bias against women, as the tech industry used to be dominated by men. Therefore, the Amazon recruiting system misunderstood that male candidates were preferred. It penalized resumes that included the “feminine” words, such as “captain of the women’s chess club.” Therefore, Amazon stopped using the algorithm for recruiting.
Many leading technology companies are working to close the gender gap in hiring. This disparity is most pronounced among technical personnel, such as software developers, where there are many more men than women. Amazon’s experimental recruiting mechanism followed the same pattern, learning to penalize resumes that included the word “woman” until the company discovered a problem.
Racial bias has been demonstrated by a health risk prediction algorithm used by more than 200 million US citizens. The algorithm predicted which patients would require additional medical care. However, it gave erroneous results that favored white patients over Black patients, because it relied on the wrong metric to determine the need for intervention: past costs.
Also, let’s not forget about the numerous examples of bias in social networks. In 2019, Facebook allowed its advertisers to intentionally target ads based on gender, race, and religion. For example, job ads favored women for nursing or secretarial positions, while job ads for janitors and taxi drivers were mostly shown to men, in particular minority men.
As the data in technical platforms is later used to train machine learning models, these biases lead to biased machine learning models. As a result, Facebook will no longer allow employers to include age, gender, or race in their ads.
The five misconceptions described in this article reveal flaws in many companies’ understanding of the current state of AI. However, these questions remain open. Indeed, as the field develops there may well be more misconceptions that arise.
For many businesses, AI is a useful tool — when applied correctly. AI can improve interactions with customers, analyze data faster, assist in decision-making, generate early warnings of upcoming disruptions, and more. It also has a number of useful applications in an industrial environment, for example, computer vision/recognition, which allows it to detect a defective part much more efficiently and quickly than a human operator. The Postindustria team can help businesses create AI-powered solutions to automate workflows and make production cycles more efficient.
Thank you for reaching out,
User!
Make sure to check for details.