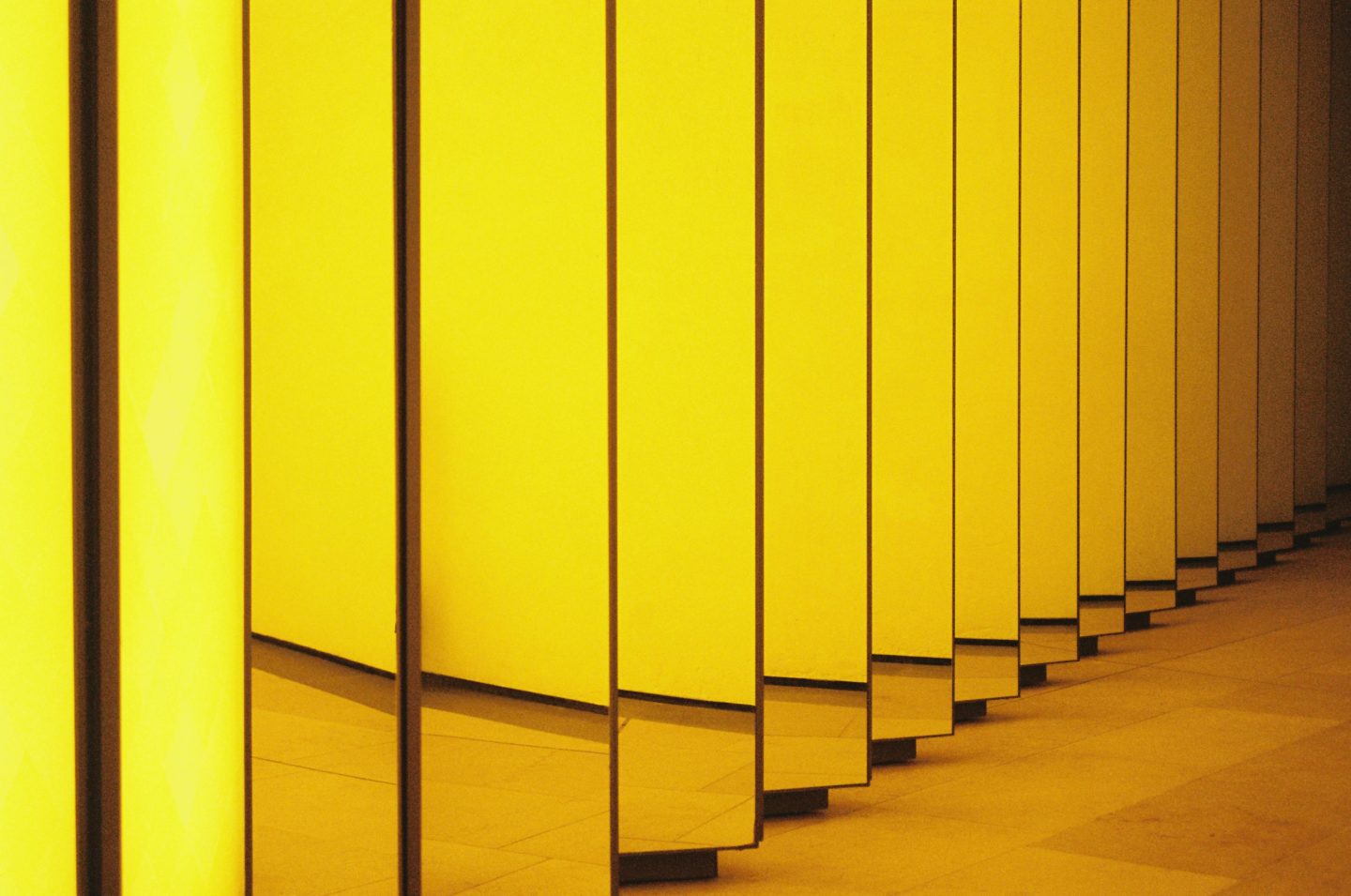
- Artificial Intelligence
- Machine learning
In the past few years, the progress achieved in artificial intelligence (AI) development prompted companies in a range of industries to adopt AI. Healthcare is one of the domains where the application of AI subsets — machine learning and deep learning — has potential to yield solid benefits to both hospitals and patients. Interest in machine learning services for healthcare projects has grown, backed by the emergence of more and more AI healthcare startups.
According to the research and consulting firm Stratistics MRC, the global healthcare AI market was valued under $1bn in 2017 and is expected to reach $19.3bn by 2026, showing 39.7% annual growth. An increased number of AI applications and advances in data analytics are two of the factors fueling market growth.
Promising to transform diagnosis and treatment of diseases, the integration of ML services into healthcare differs from its application in other domains. In this article, we discuss what’s unique about ML in healthcare and what you need to know before adding it to your healthcare project equation.
The first experiments that used machine learning services for healthcare projects date back to the 1960s. At that time, computer scientists had little patient data to use in experiments with ML algorithms efficiently. Since then, the digital revolution amassed terabytes of data that are now being used to develop ML-enabled solutions for healthcare. This wealth of data generates higher accuracy of ML models and better outcomes for patients than when ML first came on the scene.
However, integrating AI into clinical workflows still has important issues to overcome. Here are some of them:
Chronic diseases, such as cancer, diabetes, and heart disease are the leading causes of death in the US, according to the US National Center for Chronic Disease Prevention and Health Promotion. They also cost the country around $3.8 trillion in healthcare expenditures annually. This is in part due to patients’ reluctance to visit GPs in a timely manner, which could be ameliorated with ML-enabled solutions that help track patient conditions and predict disease progression.
In 2010, the McKinsey consultancy reported that an analysis of over 300 DMPs, when they were first deployed, showed no conclusive evidence of better long-term outcomes for patients or major cost savings. Asthma DMPs were even reported to have had a negative effect on health outcomes and costs. Upgrading DMPs with data-driven ML algorithms has potential to improve their efficiency.
Human errors happen everywhere. And healthcare is no exception. AI-driven tools for patient treatment are unlikely to replace clinicians anytime soon and will be rather aimed at augmenting their capacity than replacing medics. Advanced healthcare solutions relying on ML algorithms can help reduce the risk of human error.
Recent technological breakthroughs and the rising role of electronic health records (EHR) with huge amounts of patient data have enabled research in ML to develop solutions for healthcare. However, these advances are accompanied by challenges too.
The availability of big data is one of the biggest drivers of ML advances. According to Statista’s predictions, the worldwide volume of data created, captured, copied, and consumed will reach 120 zettabytes by 2023. A zettabyte is enough to store 60 billion video games or 30 billion 4K movies. In 2020, this number was slightly higher than 64 zettabytes.
The potential for big data to play a role in healthcare is evidenced by some high-profile deals that closed over the past decade. In 2015, IBM purchased a company called Merge, which specialized in medical imaging software for $1bn, acquiring huge amounts of medical imaging data for IBM. In 2018, a pharmaceutical giant Roche acquired a New York-based company focused on oncology, called Flatiron Health, for $2bn, to fuel data-driven personalized cancer care.
Algorithmic advances have also catalyzed the use of ML in healthcare. Machine learning using techniques like high-dimensional features, semi-supervised and unsupervised learning, and modern deep learning introduced solutions that can spot potentially malignant tumors in MRIs, CTs, and X-rays, analyze vital signs and predict health conditions.
The development of open-source software, like TensorFlow, Pytorch, and Theano also contributed to industry advances.
The rise of EHRs let researchers test ML algorithms and eventually implement those algorithms to analyze medical records. In 2017, 96% of hospitals in the US used EHRs, and this number is constantly growing.
However, EHR systems lack interoperability, partly because medical terminology lacks standardization, which in turn, prevents normalizing data to a standard. The challenge is that medical record systems were created for individual providers, not for exchanging records between them. Thus, the rising number of record providers and data sources creates sets of disparate low-quality data.
Heterogeneous data types is another challenge to research in this field. Data from laboratory tests, medical images, vital signs, genomics all come in different formats, making it difficult to deploy ML algorithms to all the data at once.
Another issue is wide-spread accessibility of medical datasets. At the moment, MIT claims to have the only substantially sized database of critical care health records that is publicly accessible. Its MIMIC database stores and analyzes health data from over 40,000 critical care patients. The data include demographics, laboratory tests, vital signs collected by patient-worn monitors (blood pressure, oxygen saturation, heart rate), medications, imaging data and notes written by clinicians.
Another solid dataset is Truven Health Analytics database, which data from 230 million patients collected over 40 years based on insurance claims. However, it’s not publicly available.
Heterogeneity of big data and EHR interoperability issues create roadblocks to its effective use for development of machine learning services for healthcare projects. Standardizing medical data and terminology will facilitate ML development processes and increase the accuracy of models deployed for healthcare projects.
The International Classification of Diseases (ICD-11) by the World Health Organization (WHO), the Logical Observation Identifiers Names and Codes (LOINC) database for identifying medical laboratory observations, and the US National Drug Codes (NDCs) are some of the commonly used databases for standardization of medical data and terminology.
Certain issues must be considered when deploying ML models in healthcare. Whether you want to deliver efficient solutions for early disease diagnosis, monitor health conditions, or assist with surgeries, here are some factors that make the use of ML in healthcare unique, compared to its use other industries:
Machine learning services can be a reliable and powerful tool for all kinds of healthcare projects. If we carefully address the issues mentioned above, ML has the potential to solve many challenges in healthcare. Everything comes down to choosing the right solution for your needs and implementing it in the best way possible.
That’s something Postindustria can help with. We’re skilled in custom AI development and ready to help with your medical project. We know how to build a machine learning pipeline and share expertise in medical data science. Check our healthcare projects Mahmee and Mindbloom or book a strategy session with our experts to discuss how to level up your solution.
Thank you for reaching out,
User!
Make sure to check for details.