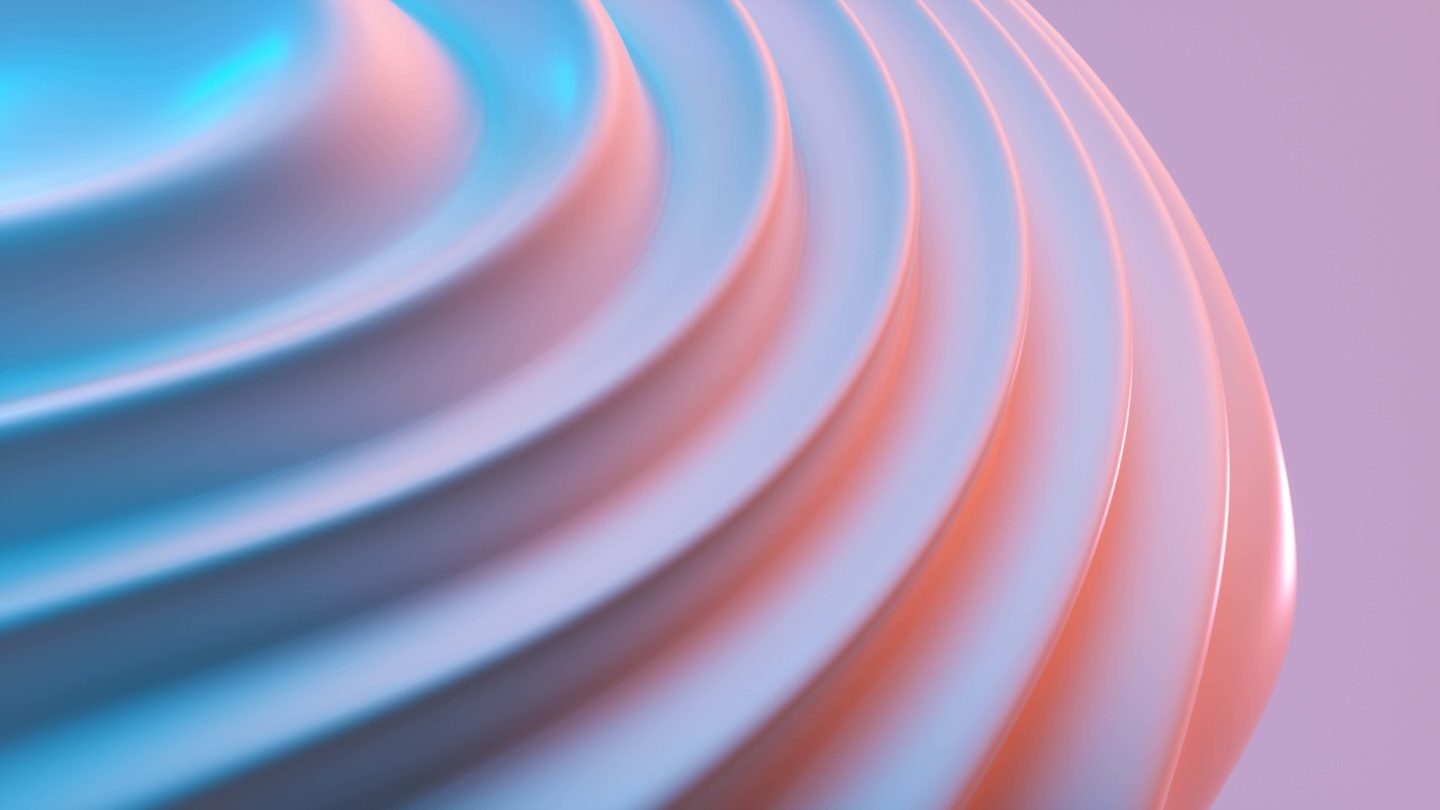
- Artificial Intelligence
Using AI Technology for Fashion Trends Prediction and Demand Forecasting
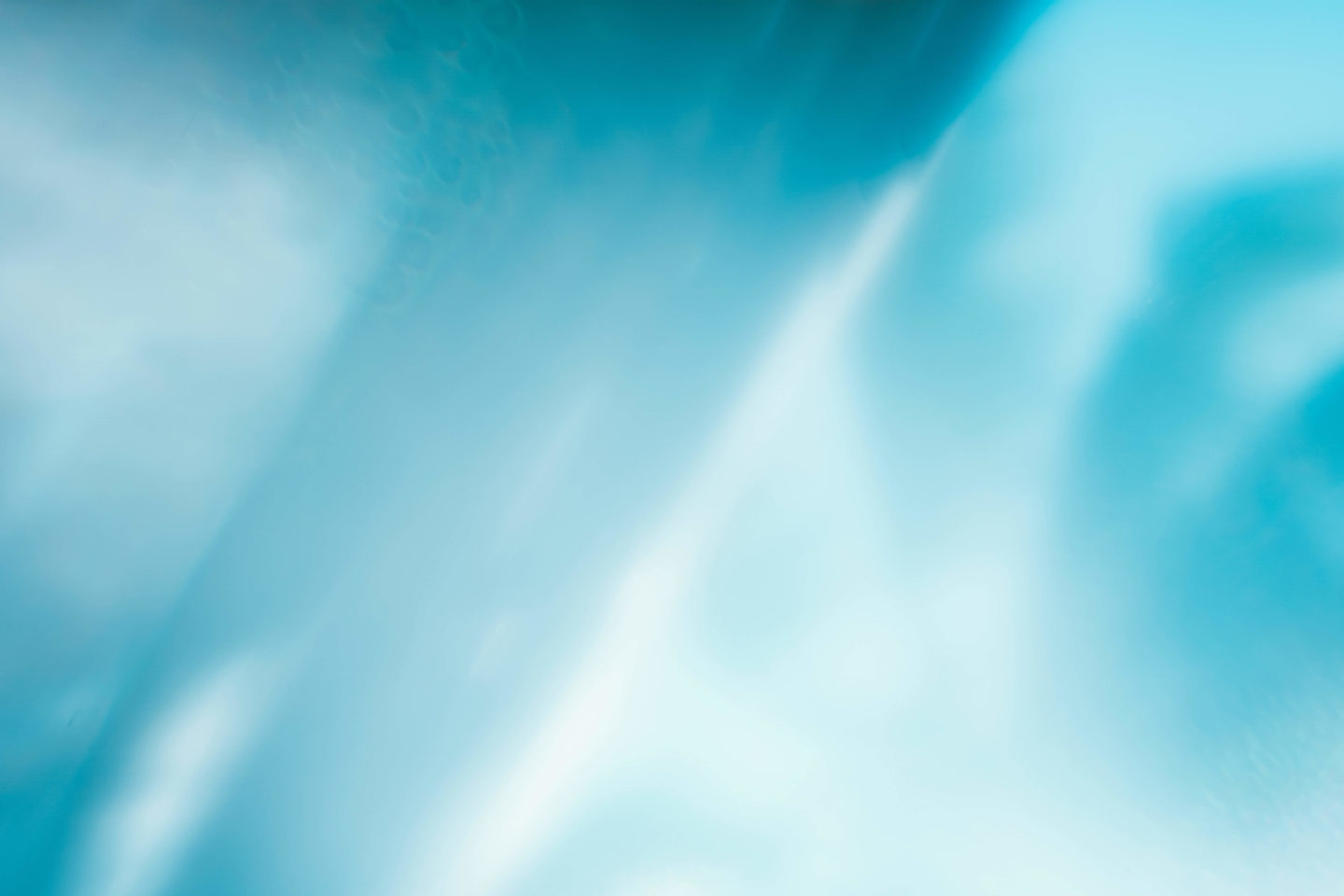
- Artificial Intelligence
AutoML Current Uses and Approaches
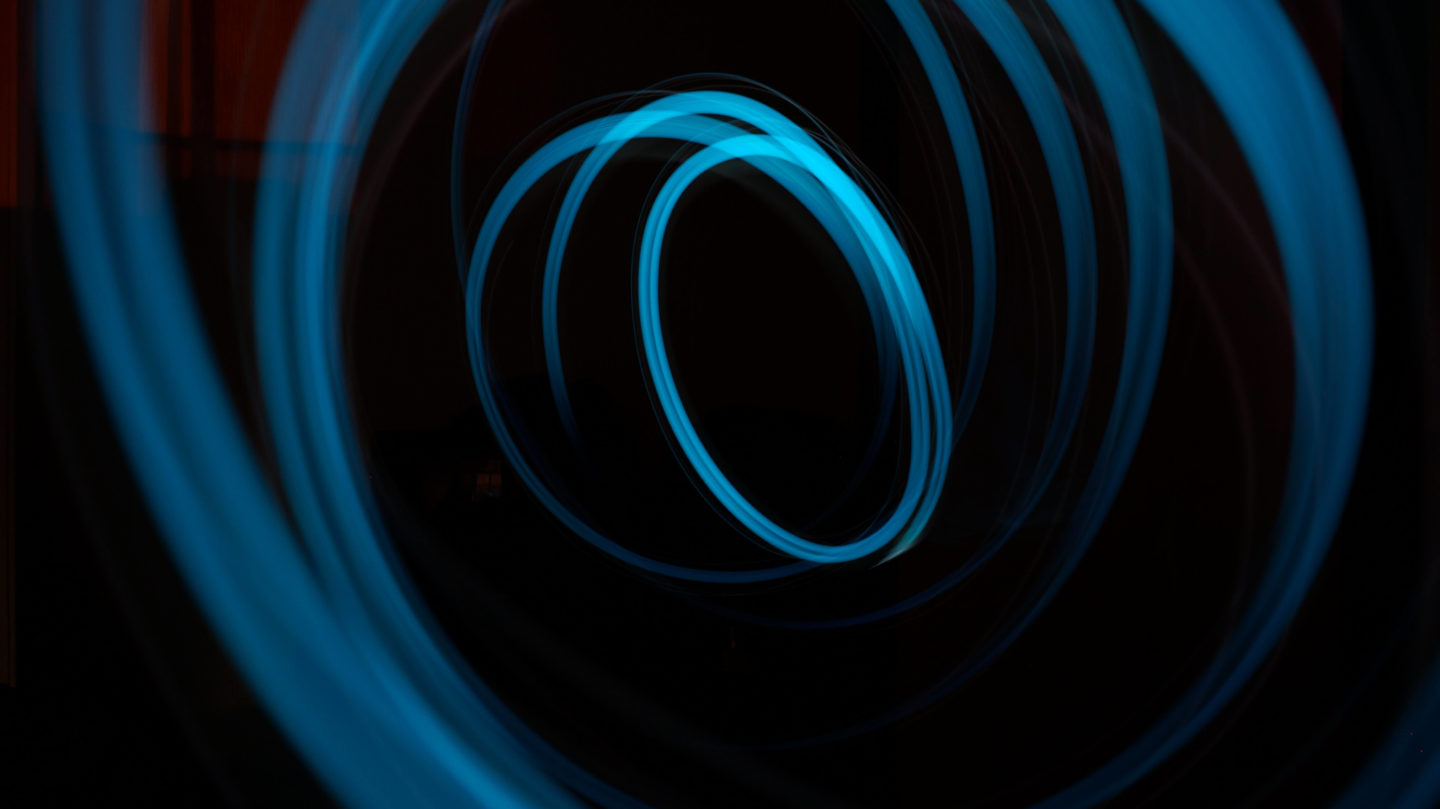
- Artificial Intelligence
The health sector generates up to 30% of global data. By 2025, health data will accumulate at a CAGR of 36%, which is faster than data from media and finance. In order to process and make sense of such vast amounts of information, the use of artificial intelligence (AI) algorithms in healthcare must increase dramatically. In this guide, we showcase how AI helps in healthcare.
With an increased number of patients in recent years, physicians process so many different kinds of data and try to understand the similarities, differences, and patterns in their patients’ disease states and symptoms.
Back in 2020, the International Data Corporation showed that 50% of hospitals were already using AI, and the other half were doing their best to implement it. Although the healthcare industry continues to adopt it, we still have a long way to go before we see AI used to its full potential in healthcare.
AI has a lot to offer the health sector. The amount of data generated in healthcare will continue to grow and this is where AI algorithms can streamline processes. For example, its ability to quickly sort through massive datasets means machine learning (ML) can help doctors devise personalized treatment plans that can mean the difference between life and death. However, few people understand that ML is only one of many AI algorithms. Let’s take a closer look at ML algorithms.
It’s not easy to answer “How can AI can help in healthcare?” before explaining the types of algorithms available. AI has branched out into many sub-disciplines; you can find out more about AI algorithms in healthcare in our recent post. Below, we describe the three most important AI algorithms currently used in the medical industry.
Machine learning. ML deals with huge datasets that must be labeled and structured before being used to train an ML algorithm. The data are stratified by an engineer using mathematical algorithms to sift through them and draw conclusions based on specified criteria.
Deep learning (DL). DL is a scalable version of ML that requires less human attention, especially during the data entry phase. It can handle larger and less structured datasets than basic ML. DL algorithms are able to independently find patterns in data.
Natural language processing (NLP). NLP is a sub-discipline of AI and ML that deals with recognizing text and speech, which are specific kinds of unstructured data.
Now that you know a little more about different categories of AI algorithms before we move on to how AI helps in healthcare, it’s important to understand the difference between the types of data that AI systems can process.
Structured data is labeled and systematized before using it as training data for an AI algorithm. For example, data stored in electronic health records, with well-defined fields and labels, is structured data. When an algorithm processes structured data, it knows exactly what is being fed to it and what result it is looking for.
Unstructured data is not organized. The AI algorithm has no identifiers (labels) or predefined structure to rely on and must figure out how to make the data useful. Examples of unstructured data include medical notes in audio or text format and images.
The ability to analyze unstructured data is one of the biggest advantages of using AI in healthcare. By applying DL algorithms, medical researchers can quickly extract valuable information from unstructured datasets.
ML involves developing basic computational models to solve problems. There are basically four types of ML: supervised learning and unsupervised learning, which we focus on here, plus semi-supervised learning and reinforcement learning.
With all the different algorithms now available, ML solutions have become more than just wishful thinking for healthcare. To learn more about types of ML, feel free to check out our post dedicated to machine learning applications in healthcare. Wherever there is complex data to process, you can count on ML to process it. Next, let’s look at some uses of ML in healthcare.
Predictive analytics. This approach uses historical data to predict future results. It recently proved its worth in the healthcare industry when the National Minority Quality Forum (NMQF) released the COVID-19 Index, a tool that predicts pandemic outbreaks. Another example of predictive analytics is using ML to quickly assess prognosis, so medical teams can target palliative consultations to high-risk individuals.
Disease identification and diagnosis. ML is able to learn from large patient datasets to identify rare diseases that are difficult for doctors to diagnose and diseases that are difficult for doctors to diagnose, particularly in their early stages. For example, the Massachusetts Institute of Technology (MIT) has developed a revolutionary DL model that can predict the onset of breast cancer years into the future.
Medical imaging control. ML significantly improves the efficiency of medical professionals by using algorithms to process complex visual information. One noteworthy application is the analysis of images to detect skin cancer. According to the paper published in the Journal of Medical Internet Research, without medical imaging, dermatologists are only 65-80% accurate. With imaging supporting their trained eye, the accuracy rate increases to 75-84%.
Robotic patient-support tasks. Robots are now used to improve patient care. Mabu, for example, is a robot making headway in providing home care without a doctor present. This yellow robotic machine helps people cope with the physiological symptoms and psychological barriers that make it difficult to treat certain chronic diseases.
Personalized consultations. To provide individualized prognoses, practitioners sometimes correlate information from electronic health records with genetic data. This process is very time-intensive. Using datasets at a scale that is beyond human capabilities to process, machine learning is paving the way for precision medicine. This new approach analyzes complex datasets and integrates multimodal data to provide individualized outcome predictions for patients.
Communication with patients. Regular screening could prevent about 1.7 million new cancer cases per year in the US. Effective outreach marketing directed to individual patients can encourage people to participate in these wellness visits. Machine learning plays a role in helping practitioners test the effectiveness of, and improve their outreach programs.
As you can see, ML is widely used in the medical industry and is already producing stunning results. You can find more information about healthcare use cases for machine learning in our article. However, ML is far from the only way to use AI in healthcare.
Artificial intelligence can positively impact medical practice by improving its three main components:
Let’s take a look at some of the most prominent use cases of AI in healthcare.
One of the greatest examples of applying deep learning in healthcare is detecting melanoma. Melanoma, the most dangerous form of skin cancer, accounts for nearly three-quarters of skin cancer deaths despite being less common. The American Cancer Society forecasts that about 207,390 new cases of melanoma, including stage zero, will be diagnosed in the US by 2022.
Deep learning shows promise for detecting melanoma and reducing mortality rates. It involves training neural networks to perform image, text, and sound classification tasks. Thanks to advances in neural network algorithms, deep learning can now compete with human accuracy in visual detection tasks. More specifically, convolutional neural networks (CNNs), roughly mimic human vision and are used to classify melanoma images. A CNN analyzes input images and ranks the importance of different objects or aspects of objects in the image to distinguish between them. Detecting patterns and parsing them makes CNNs extremely useful for image analysis
Pre-trained CNN algorithms can detect suspicious pigmented lesions (SPL) on the skin on par with dermatologists. Based on over 20,000 wide-angle images from 133 patients, MIT scientists trained a CNN algorithm to detect SPLs in primary care settings. The trained CNN analyzes SPLs in images to determine how suspicious each lesion looks, then classifies them using labels.
Early detection makes melanoma more treatable, and in many cases, completely curable. The time factor is very important in diagnosing this type of cancer. Applying deep learning to melanoma detection can speed up diagnosis, thereby making timely treatment possible. If you’d like to read more about using CNNs to detect melanoma, feel free to check out our article about the application of deep learning in healthcare.
Currently, histopathologists analyze images of tissues or cells visually. This process is time-consuming and error-prone. Machine learning can help by automating the process, making it faster and more accurate. Our experts researched this topic and prepared an article about opportunities in histopathology provided by ML image analysis.
Machine learning methods can be divided into two categories, depending on the availability of labeled data. Algorithms that use labeled data are called “supervised” learning algorithms, and those that use unlabeled data are called “unsupervised” learning algorithms.
Supervised learning algorithms infer a function that can match new input images to prelearned labels. Like other ML models, these algorithms excel at classification. For example, after training on labeled input data, supervised learning algorithms can examine a new set of images and identify characteristics that indicate cells are cancerous. The most common supervised algorithms are neural networks, support vector machines, random forests, decision trees, k-nearest neighbor, and naive bayes classifiers.
Unsupervised learning algorithms find patterns in unlabeled data. These algorithms are used in data clustering to find hidden structure in data. One of the most common algorithms is k-means clustering.
While there are still obstacles to the wide adoption of machine learning in medical image analysis, MedTech companies are actively experimenting with different methods in several areas of medicine. Below are some examples.
Computer-assisted diagnostics (CAD) uses machine learning techniques to analyze histopathological images. CAD assists with histological image analysis to detect and classify carcinoma. In particular, it has been used for the early diagnosis of breast cancer — it provides histopathologists with a reliable second opinion.
Content-based image retrieval (CBIR) searches a database and retrieves images that are similar to an example image. CBIR uses supervised and unsupervised machine learning techniques such as image dimensionality reduction as well as deep learning techniques. It retrieves images that match the example with great accuracy and speed.
Clinicopathological relationship analysis helps find correlations between the morphological features of cells and their behavioral features. An example might be the relationship between the biological features of stromal breast cancer identified in a histopathology image and how that cell metastasizes. Finding relationships helps evaluate tumors, plan treatment, and make prognoses.
Today, machine learning is not widely used in the field of histopathology, but successful cases are have been documented. A hospital in Seoul reported that the accuracy of their diagnoses increased from 83% to 87% thanks to ML. The growing world population is expected to increase demand for these technologies. Therefore, investing in machine learning makes sense for progressive organizations and medical technology start-ups.
According to Allied Market Research, the global computer vision industry will quadruple over the next 10 years, growing from $9.45 billion in 2020 to $41.11 billion by 2030.
Computer vision is a form of AI that uses algorithms to replicate human visual recognition abilities. The most common applications of computer vision in healthcare are in medical imaging, helping physicians identify pathologies and diseases using x-rays, computed tomography, and magnetic resonance imaging. Now let’s take a closer look at how computer vision can transform healthcare.
Radiological diagnostics. The ability of computer vision to detect patterns in images, including x-rays, CT scans, and MRI images can help doctors diagnose a variety of diseases more accurately and in a more timely manner than manual inspection images. Deep learning-enabled solutions in this area are based on neural network models trained to classify medical images. They can detect lesions, tumors, and suspicious spots that require further examination.
Medical imaging. Medical imaging (non-radiological) is another area where computer vision can contribute to the early detection of disease. In this case, imaging allows doctors to more comprehensively examine a patient’s tissues and organs to detect abnormalities. The Arterys medical imaging AI platform, for example, offers hospitals and medical imaging centers software to help detect abnormalities in the heart, lungs, and brain.
Tracking postoperative blood loss. Computer vision can also reduce postoperative mortality. For example, it can be used to track patient blood loss in real-time. Doctors usually assess blood loss visually, which is not always accurate. During childbirth, inaccurate assessments can lead to a deterioration in a woman’s condition, and even death. Using computer vision systems to track blood loss can reduce maternal mortality due to undetected bleeding. California-based medical technology company Gauss Surgical developed Triton, an AI-based monitoring solution that helps doctors accurately assess blood loss after childbirth, including after cesarean section. To learn more about how computer vision is transforming the industry, read our more detailed discussion in this article about computer vision in healthcare applications.
The main driving force behind the emergence of computer vision in radiology has been the desire for greater efficiency and effectiveness in clinical care. Technological advances, especially in ML, will change radiology practices. And that’s not limited to interpreting images. Machine learning will shape the entire data flow — from ordering an X-ray or MRI to making a diagnosis.
ML is rapidly advancing and could become consistently better than doctors at image analysis very soon. However, the best systems currently available are roughly equivalent to humans and are used only for research purposes, and here’s why:
According to an IBM study, ML models can achieve high accuracy and can already be on par with medical professionals. The question of whether diagnoses based solely on ML are acceptable is already an ethical issue. Our engineers expressed their opinion on why computer vision in radiology will not replace doctors for a long time and you can read about it in our recent post.
AI is a set of methods, tools, and algorithms that power systems to mimic human cognition. When someone mentions AI in healthcare, most often they are referring to machine learning.
Since 2016, AI has made a significant leap in usage. According to the 2021 AI100 report, AI is currently undergoing active testing in medical centers for diagnosis, symptom prediction, and research, including drug discovery. Here are some of its most promising use cases:
Read more about how far the current state of AI is from realizing its potential in our post about AI challenges in healthcare.
There are reasonable barriers to AI’s implementation in clinical practice. First, there’s a lack of quality medical data. Clinicians need high-quality datasets to validate AI models, both technically and clinically. However, obtaining patient records and images to test algorithms is difficult because medical data is fragmented across different electronic health records and software platforms.
The metrics used to measure the performance of AI models are not always useful for clinical applications. This is known as the AI chasm — the gap between the technical accuracy of AI algorithms and clinical performance in the real world. In addition, research on AI in healthcare suffers from a lack of standardized methodology, prospective studies, and peer-reviewed data.
AI-based systems with machine learning models can have unreliable results if the data used to train them is biased. Bias may be related to patient demographics such as race, gender, and socioeconomic factors, or to researcher bias in algorithm design and choice of training datasets. We addressed this topic in our post about AI bias in healthcare.
The use of AI in healthcare, in terms of software solutions, equipment, and services, is predicted to continue growing year over year. In 2021, its estimated value was $10.4 billion, and it’s expected to hit the $120.2 billion mark in 2028. This predicted compound growth rate of 41.8% is due to the following factors:
The potential of AI in healthcare is tremendous, which makes it one of the most attractive niches for investment. The US and China are currently the countries with the highest amount of venture capital spending on medical AI. Feel free to check this article that explains where healthcare AI solutions are already being applied.
A survey conducted by KPMG showed that over the next two years, healthcare organizations will prioritize investment in AI in medical technology. Statista’s 2021 survey of US healthcare business leaders found that 40% of respondents find healthcare AI solutions more valuable than they expected.
Medical robotics is another medical technology that’s advancing. It’s a trend that will dominate the industry in 2022 and beyond. Robots in healthcare assist during surgeries, help patients recover during rehabilitation, facilitate sterilization and delivery of equipment in hospitals, diagnose conditions, and improve telemedicine.
According to the 2021 Fortune Business Insights forecast, virtual reality (VR) in the healthcare market is also expected to grow. VR technology benefits both healthcare professionals and hospitals. It’s used to help treat patient conditions, educate medical professionals, and assist surgeons.
We discussed these and several other trends in our article about medtech trends, 2022.
Sophisticated AI models aren’t yet ready for mass deployment because of data bias, transparency issues, and privacy protection concerns, but all of these challenges are solvable. More importantly, the potential benefits of AI-enabled systems far outweigh the effort needed to perfect them and solve the challenges.
AI presents exciting opportunities to improve healthcare diagnostics, early prediction of symptoms, and drug development. It can help doctors see a complete picture of their patients and consider alternative diagnoses and treatment options. On top of that, current AI- and ML-based systems are already helping companies improve their workflow.
Postindustria can empower you with advanced AI development solutions, including natural language processing, big data analytics, and robotic process automation. Reach out to our team to see how we can bring your ideas to life!
Thank you for reaching out,
User!
Make sure to check for details.